The 3 Day GenAI Creator Workshop for Kids in the US: An Initiative by TTA, Powered by {igebra.ai}
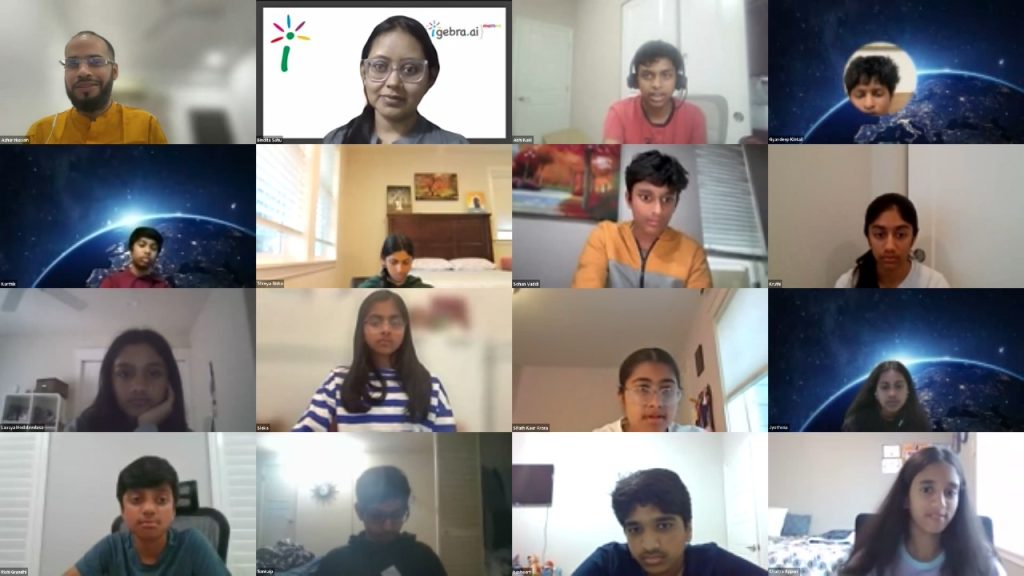
In the vibrant tapestry of technological education, a remarkable initiative woven by the Telangana American Telugu Association has captured the imagination of young minds across the United States. This visionary project, executed with flair by {igebra.ai}, a U.S.-based AI research, development, and education powerhouse, took the form of the GenAI Creator Workshop. Held from May 3rd to 5th, 2024, this free, online workshop opened a portal for children to explore the fascinating world of generative AI by creating songs and 3D animations. It was not merely a course but an adventure into the limitless potential of AI, designed to ignite curiosity and foster innovation among the leaders of tomorrow. The workshop’s core mission was to peel back the curtain on the mysteries of generative AI, enabling children to harness these advanced tools to create something truly unique. Beyond the technical skills, the program sought to plant seeds of inspiration, encouraging participants to ponder the latest tech advancements and consider their future roles in shaping this dynamic field. It was an invitation to dream big, envisioning new possibilities that extend beyond the current horizons of technology. The workshop catered to two distinct groups: Group A for elementary school students and Group B for middle and high school students. Each group participated in three meticulously planned workshops focusing on different aspects of generative AI tools and their applications. For instance, Group A was introduced to video song creation using tools such as Suno AI, Midjourney, RunwayML, and ClipChamp. In contrast, Group B explored the creation of animated video stories through platforms like ChatGPT, Midjourney, Pika Labs, Eleven Labs, and ClipChamp. These sessions were supplemented by support sessions to assist participants in completing their projects, ensuring a hands-on, supportive learning environment. Following the enlightening workshops, the participants were tasked with submitting their creations for the first round of competition. This exciting phase was designed to showcase the skills and creativity they had honed during the workshops. From this initial submission, twelve semi-finalists were selected to advance to the grand finale. This culminating event will take place in a hybrid format, combining both virtual and in-person elements, at the prestigious Seattle Convention Center on May 25th and 26th. This hybrid approach ensures that all participants, regardless of their location, have the opportunity to engage and showcase their innovative projects to a wider audience. The true spirit of the workshop was reflected in the enthusiastic collaboration among participants, mentors, and organizers. It was a collective endeavor that deserved a standing ovation, with parents and mentors playing crucial roles in nurturing the talents and ambitions of these young creators. Special recognition goes to Vamshi Reddy and the Telangana American Telugu Association Team, whose support was instrumental in bringing this idea to life. Under the guidance of visionaries like Kalyan Kaki and the expert chairmanship of Avinash Gupta Kolluri, the workshop was steered towards success. The proactive co-chairs, Sudhir Dachepalli, Praveen Paluri, and Naresh Kumar Penugonda, along with the GenAI Extended Team members like Kiran Kaki and Satish Chigullapally, contributed significantly to the smooth execution of the event. The wisdom of the judging panel, including Ganesh Gella and Praveen Kashimsetty, enriched the learning experience, providing critical feedback and insights. A round of applause is also due for the dedicated {igebra.ai} team including Srini Vemula, the founder of {igebra.ai}, Chiranjeevi Maddala, Director Product Development & Marketing, for their unwavering support with trainers Azhar Hussain and Karuna Jain, whose creativity and commitment were pivotal in nurturing the next wave of tech innovators. The GenAI Creator Workshop stands as a beacon of innovation and education, preparing our children for a future where they not only adapt to new technologies but also drive them. As we applaud the achievements of these young creators and their mentors, we look forward to more such initiatives that foster creativity and technological fluency among the future stewards of our world. Let’s continue to celebrate and support such transformative educational endeavors that promise to shape a brighter, more inventive future.
Best Strategies for the Responsible Use of Generative AI in Education
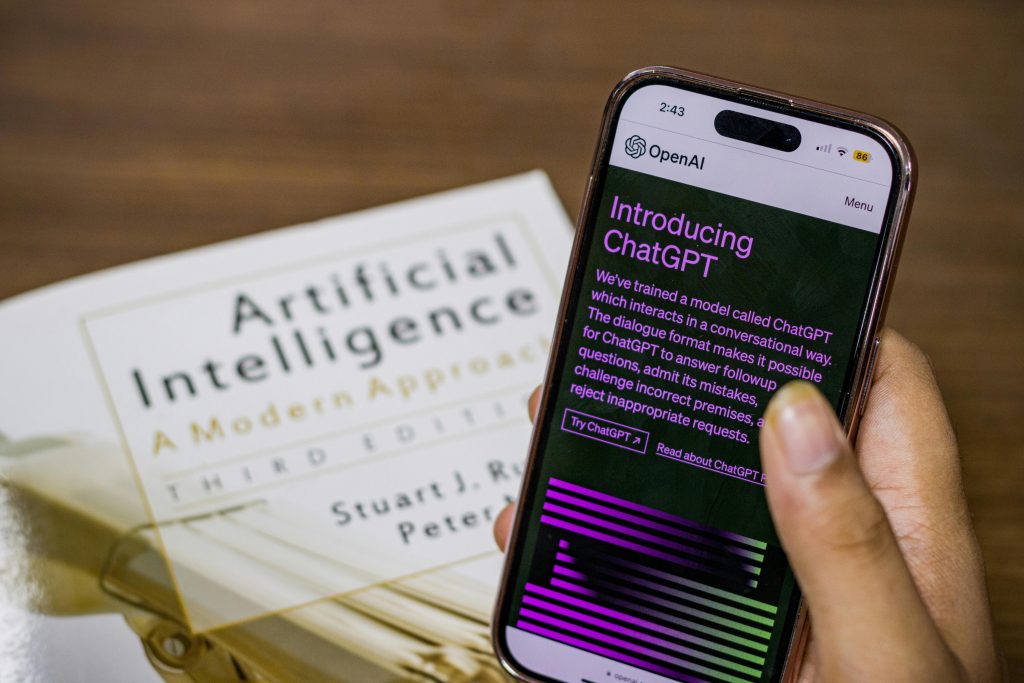
Employing Generative AI in education can enhance learning outcomes, strengthen teacher performance and wellness, and foster equity in educational practices. Conversely, lacking such oversight, there exists the potential for privacy violations, inconsistent disciplinary procedures, and inefficient application of AI technologies in educational settings. What is Generative AI? Generative AI refers to a subset of artificial intelligence technologies that can generate new content, ranging from text and images to music and code, based on the patterns and information it has learned from training data. This technology utilizes advanced machine learning models and algorithms, particularly those based on neural networks such as Generative Adversarial Networks (GANs) and transformer models like OpenAI’s GPT (Generative Pre-trained Transformer) series. How Generative AI Enhances Learning Challenges and Ethical Considerations of Using Generative AI in Education 7 Strategies for Responsibly Using Generative AI in Education Here are seven Strategic guidelines that educators, policymakers, and educational leaders should consider when establishing protocols to ensure the responsible and effective implementation of AI in education. These seven Strategies offer a structure for the responsible and effective use of AI in education. By following these guidelines, educators and policymakers can take advantage of AI’s benefits while tackling its challenges, ensuring an approach that is balanced, ethical, and inclusive. Conclusion As we navigate the evolving landscape of education technology, it’s clear that AI holds transformative potential for enhancing learning environments, personalizing educational experiences, and streamlining administrative tasks. However, the integration of AI into education comes with its own set of challenges and ethical considerations, particularly regarding data privacy, equity, and the preservation of human oversight. By adhering to the strategic guidelines outlined in this blog, educators and policymakers can ensure that AI is used responsibly and effectively. As AI technologies continue to develop, it will be crucial to remain vigilant and adaptable, continuously assessing their impact and refining their use to serve the best interests of all students. Embracing AI in education is not just about leveraging new tools, but about fostering an inclusive, equitable, and enriched learning landscape for future generations.
Generative AI’s Influence on Robotics
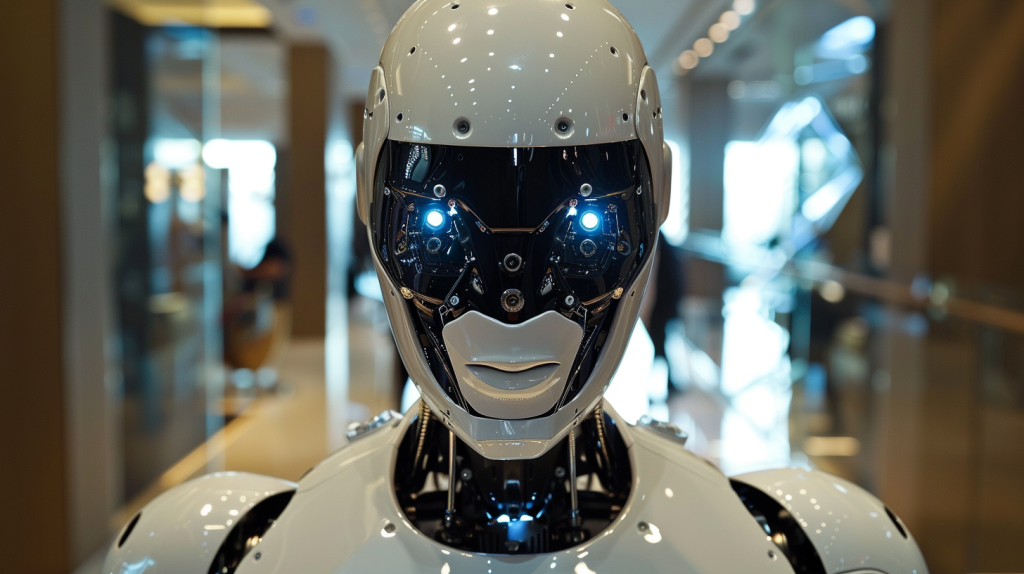
Robots are getting a massive upgrade thanks to the rise of generative AI. This cutting-edge technology is giving our mechanical friends unprecedented smarts – allowing them to see, learn, adapt, and even create in wildly humanlike ways. At its core, generative AI uses deep learning models to process vast pools of data and then generate brand new, realistic outputs like images, text, audio and more. When integrated into robotics systems, this AI can supercharge capabilities across the board. Imagine robots that can instantaneously map their surroundings in photorealistic 3D, plan optimal movements with precision choreography, or even hold flowing conversations. With generative AI, they can perceive their environment like never before, acquire skills independently through experiences, and seamlessly interface with humans. This game-changing tech is already revolutionizing fields like manufacturing, healthcare, and space exploration. Autonomous robots can take on increasingly complex tasks, navigating unpredictable settings while continuing to learn and evolve. The market’s rapid expansion reflects generative AI’s immense potential. Researchers predict this space will swell from $7.9 billion in 2022 to $42.7 billion by 2027 at a blistering 40% annual growth rate. So get ready to witness AI-powered robots that challenge what we thought possible from machines. With their generative intelligence unleashed, the future of robotics has been revolutionized. What Are AI-Powered Robots? Forget those clunky old robots – the new breed is bordering on bionic. AI-powered robots are like cyborgs, fusing advanced sensors with artificial intelligence to create hyper-aware, quick-witted machines. They’re packing vision that sees in 3D, vibration detectors that sense the slightest movements, and environmental scanners to map their surroundings in real-time. Feed all that data into their AI brain, and these robots can instantly comprehend their world, identify objects and threats, and dynamically decide how to operate. From factories to hospitals, these smart part-man-part-machine robots are changing what’s possible through their uncanny awareness and decision-making prowess. Difference between Traditional Robot and AI-Powered Robot Aspect Traditional Robotics AI (Artificial Intelligence) Definition Machines designed to physically interact with the world. Programs designed to process data, make decisions, and learn from experiences. Primary Function Performing physical tasks such as assembling products or performing surgeries with high precision and efficiency. Analyzing data, identifying patterns, and making complex decisions dynamically. Examples Assembly line robots constructing cars, surgical robots performing procedures. Systems guiding surgical decisions, algorithms for trading stocks on Wall Street. Characteristics Known for physical capabilities, excelling in repetitive, high-speed tasks to boost productivity. Known for cognitive capabilities, versatility in processing information and making informed decisions. Origins Emerged from the industrial age, with the term “robot” coined in 1921, focusing on refining physical design. Conceptualized in 1956, with rapid evolution driven by theoretical advancements and implementations by startups. Role in Integration Serves as the “body” that executes tasks. Acts as the “mind” that plans, perceives, and controls. Future Outlook Increasingly incorporating AI to become more autonomous and efficient. Continues to evolve, increasingly integrating with robotics to enhance both cognitive and physical capabilities. Current Landscape of AI-Powered Robotics The present landscape of AI and robotics is marked by unprecedented advancements. Machine learning algorithms, deep neural networks, and sophisticated sensors have propelled AI to new heights, enabling machines to understand, learn, and make decisions with increasing autonomy. In tandem, robotics has evolved beyond traditional industrial applications, embracing collaborative and adaptive technologies. HD Atlas (Boston Dynamics) Figure 01 Figure 1 is a humanoid robot developed by Figure in collaboration with OpenAI, are diverse and cutting-edge. Phoenix (Sanctuary AI) Phoenix (Sanctuary AI) is designed for highly interactive environments, Phoenix likely incorporates advanced AI to manage complex interactions and tasks. Phoenix stands at 170 cm and can carry up to 25 kg. Equipped with 20 DoF robotic hands for precise manipulation Runs on Sanctuary AI’s Carbon AI control system. Mimics human behavior and understands natural language Can learn new tasks in 24 hours. Improved visual acuity, tactile sensing, and training data capture Observes, assesses, and acts on tasks efficiently. Supports human supervision, fleet management, and remote operation Reduced task automation time from weeks to 24 hours. Improved hardware, software, uptime, and build time for increased flexibility and durability Digit (Agility Robotics) Digit (Agility Robotics) known for its versatility in navigation and carrying abilities, making it suitable for logistics and delivery applications. Atlas (Boston Dynamics) Atlas (Boston Dynamics) is a well-known figure in robotics, capable of running, jumping, and performing complex maneuvers, often used in research and rescue operations. H1 (Unitree) H1 (Unitree) is a part of a new generation of humanoid robots focusing on cost-effective designs, aiming to make robotic technology more accessible. Optimus Gen 2 (Tesla) Optimus Gen 2 (Tesla) is newer iteration in Tesla’s line of robots, potentially focusing on manufacturing and general-purpose tasks, emphasizing Elon Musk’s vision of a highly versatile utility robot. Components of AI Powered Robot Groundbreaking Applications of AI in Robotics AI in robotics aims to create an intelligent environment in the robotics industry for better automation. It uses computer vision techniques, intelligent programming, and reinforced learning to teach robots to make human-like decisions and execute tasks in dynamic conditions. We’ll cover the following use cases of AI in robotics: Category Robot Type Benefits Manufacturing Quality Control Improved product quality, Reduced human intervention Collaborative Robots Improved safety, Boosted productivity Autonomous Robots Increased human safety, Improved precision Assembly Robots Accident and injury prevention, Workflow optimization Aerospace Autonomous Rover More efficient Mars’ surface research, Increase object identification Robotic Companions Improved work experience and efficiency for astronauts Advanced Drones More successful and safer rescue missions, Better effort prioritization Transportation and Delivery Advanced Drones Improved navigation and route planning, Increased delivery speed Agriculture Advanced Drones Optimized crop yields, Reduced waste, Reduced labor costs Healthcare Robotic Assistants Improved patient care, Performance increase for medical staff Robotic Surgery Reduced risk of complications for the patient, Greater precision Service Robots Personalized patient experience, Improved patient’s emotional state Customer Service Social Robots Increased customer engagement and loyalty Service Robots Increased product and service delivery speed MilTech Unmanned Aerial Vehicles Safer reconnaissance and surveillance, Efficient bomb disposal
Unleashing the Revolutionary Impact of Generative AI in Gaming Industry
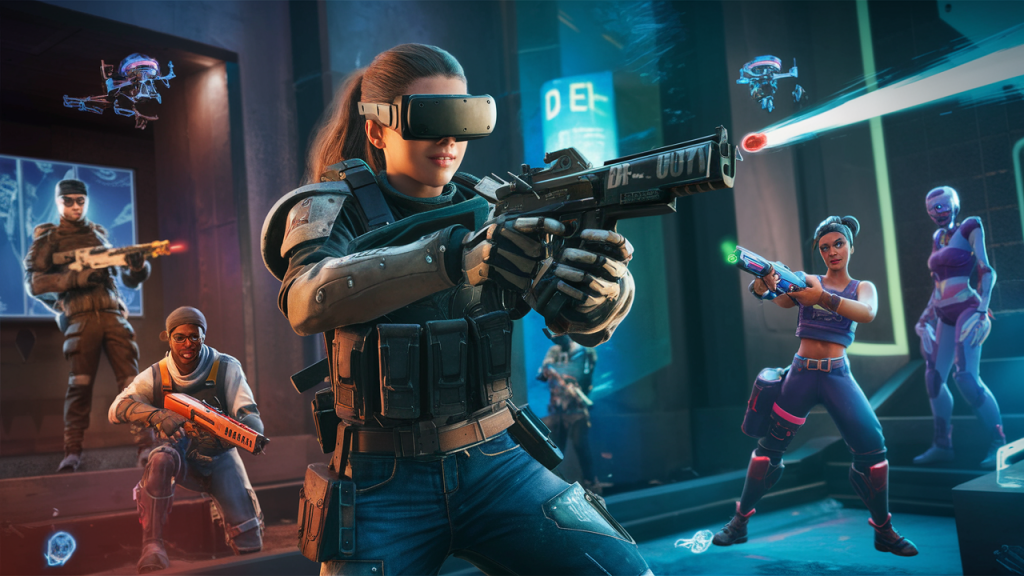
Gamers, brace yourselves! The gaming landscape is undergoing a monumental shift with generative AI, a groundbreaking technology revolutionizing every facet from world-building and character development to narrative depth. Generative AI is transforming how we play, create, and engage within virtual worlds, ensuring each gaming session is a unique, unforgettable adventure. As generative AI continues to evolve rapidly, it promises to unlock extraordinary possibilities within the gaming industry, making every playthrough thrilling. How Generative AI is Used in Gaming Industry Transformative Effects of Generative AI on the Gaming Industry From simple beginnings to complex systems, the gaming industry has embraced generative AI, ushering in a new era of creativity and innovation. This cutting-edge technology is not just enhancing but revolutionizing the way games are conceived, developed, and played. Most Popular AI Games Artificial intelligence has revolutionized the gaming industry, giving rise to a diverse range of video games that leverage AI to enhance player experience, create dynamic environments, and develop complex game mechanics. Below are some of the top video games that effectively incorporate artificial intelligence, each offering unique challenges and immersive gameplay: These games showcase the incredible potential of AI in creating more engaging, challenging, and personalized gaming experiences, and they represent just the beginning of AI’s impact on the gaming industry. Advantages of Generative AI in Gaming Industry Disadvantages of Generative AI in Gaming Industry These pros and cons highlight the transformative impact of generative AI on the gaming industry while also underlining the need for careful consideration and management of the technology to harness its benefits fully and responsibly. Future of AI in Gaming Industry Leaving You With This In conclusion, generative AI is revolutionizing the gaming industry, enhancing how games are designed, developed, and played. This technology brings immense benefits, including heightened creativity, personalized experiences, and cost efficiencies. However, it also presents challenges such as ethical concerns, intellectual property issues, and potential impacts on jobs. As we look to the future, the industry must balance innovation with responsibility, ensuring that AI’s integration into gaming remains beneficial and inclusive for all stakeholders. The potential for generative AI in gaming is vast, promising a future where the virtual landscapes are as limitless as the technology itself. Embracing this future means fostering creativity and continuously enriching the gaming experiences for players globally.
Deep Dive into Neural Networks: The Backbone of Generative AI
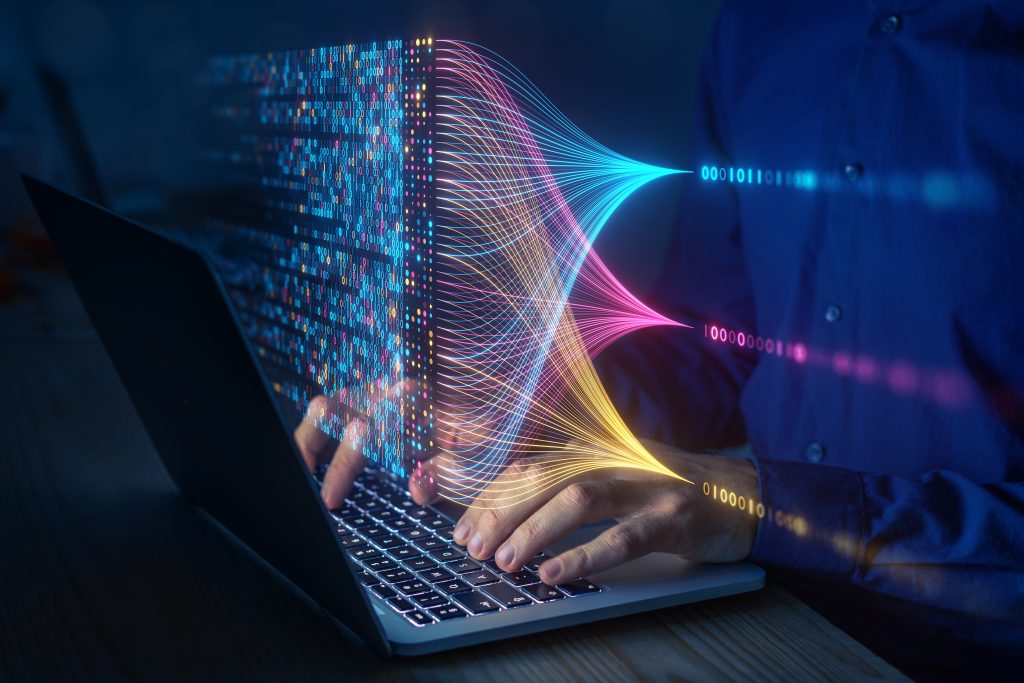
Introduction to Neural Networks and Generative AI Neural networks are pivotal in the field of machine learning and artificial intelligence, inspired by the architecture and functionality of the human brain. These complex computational models, comprising interconnected nodes or artificial neurons, mimic the way biological neural networks process and transmit information. The Power of Generative AI Leveraging Neural Networks Generative AI is a cutting-edge field that utilizes neural networks to create entirely new content, from text and images to audio and video. By digesting vast datasets and detecting underlying patterns, these AI models can generate outputs that often exceed the quality of human-generated content. Unleashing Creativity One of the most exciting applications of generative AI is its ability to spur creativity. These technologies assist artists in creating unique artwork, composing original music, and writing engaging stories, thereby pushing the boundaries of human creativity. Revolutionizing Education Imagine an educational system where learning is customized to the needs of each student. AI-powered tutors could generate tailored lessons and practice materials dynamically, making education more adaptive and ensuring no student is left behind. Enhancing Accessibility Generative AI also enhances accessibility, producing real-time captions, audio descriptions, and translations that make content more accessible to people with disabilities, and creating alternative formats of educational materials to foster inclusive learning. Milestones in the Evolution of Neural Networks 1943: McCulloch-Pitts Model: The first mathematical model of an artificial neuron, laying the foundation for neural network research. 1958: Perceptron: The development of the perceptron, the first practical neural network algorithm, by Frank Rosenblatt. 1969: Limitations of Perceptron: The Minsky-Papert book highlighting the limitations of the perceptron, leading to a decline in neural network research. 1986: Backpropagation Algorithm: The introduction of the backpropagation algorithm, reviving interest in neural networks and enabling more complex architectures. 1997: Long Short-Term Memory (LSTM): The development of LSTM, a type of recurrent neural network capable of learning long-term dependencies, by Sepp Hochreiter and Jürgen Schmidhuber. 2006: Deep Learning Breakthrough: The breakthrough in deep learning by Geoffrey Hinton and his team, demonstrating the power of deep neural networks. 2012: AlexNet and ImageNet: The success of AlexNet, a deep convolutional neural network, in the ImageNet Large Scale Visual Recognition Challenge, showcasing the potential of deep learning for computer vision. Exploring Types of Neural Networks 1. Feedforward Neural Networks: These are the simplest type of artificial neural network architecture. In this design, the information moves in only one direction—forward—from the input nodes, through the hidden layers (if any), and to the output nodes. There are no cycles or loops in the network. FNNs are widely used for pattern recognition and classification tasks because they are straightforward and effective at processing a variety of input types. 2. Recurrent Neural Networks (RNNs): These networks are distinguished by their “memory” as they process sequences of inputs by maintaining a state or memory of previous inputs in their internal layers. This is achieved through loops within the network that allow information to persist. RNNs are particularly powerful for tasks that involve sequential data, such as time series analysis or natural language processing. 3. Convolutional Neural Networks (CNNs): These networks are specifically designed for processing grid-like data such as images. CNNs use convolutional layers that apply convolutional filters to the input to create feature maps that summarize the presence of detected features in the input. These networks have been instrumental in pushing forward the field of computer vision by enabling high accuracy in tasks such as image and video recognition, image classification, and medical image analysis. 4. Long Short-Term Memory networks: There are a special kind of RNNs capable of learning long-term dependencies in data. They were designed to avoid the long-term dependency problem typical RNNs face, where it becomes difficult to learn connections from early data points in a sequence to later ones. LSTMs have a chain-like structure, but the repeating module has a different structure: instead of having a single neural network layer, there are four, interacting in a very special way. 5. Generative Adversarial Networks: It consist of two neural networks, a generator and a discriminator, which contest with each other. The generator learns to generate plausible data, while the discriminator learns to distinguish genuine data from fake data produced by the generator. This setup enables GANs to generate high-quality, realistic synthetic data. 6. Modular Neural Networks: It consist of multiple different networks that function and learn independently to solve distinct parts of a problem. Each module specializes in different aspects of the overall task, and their outputs are combined to produce the final output. This division of labor can often lead to more efficient learning and better generalization capabilities. Each of these neural network architectures plays a critical role in advancing various AI applications and solving complex problems that involve vast amounts of data and require sophisticated pattern recognition and learning capabilities. How Neural Networks Learn Neural networks adjust the connections between neurons through a process called training, where they are exposed to large datasets. This allows them to identify patterns and optimize parameters to enhance prediction accuracy or generate innovative outputs. Architectural Complexity of Neural Networks To truly appreciate the power of generative AI, it’s essential to understand the architectural complexity of neural networks. These systems are composed of multiple layers, each responsible for different computations and transformations: Input Layer: This layer receives the raw data, such as text, images, or numerical values, and prepares it for processing by the subsequent layers. Hidden Layers: These layers perform the bulk of the computations, extracting features and patterns from the input data. The depth and complexity of these hidden layers determine the network’s capability to handle intricate tasks. Output Layer: This final layer generates the desired output, whether it’s a classification, prediction, or in the case of generative AI, a newly created piece of content. Diverse Applications of Artificial Neural Networks Chatbots and Natural Language Processing (NLP) Financial Forecasting Optimizing Logistics and Supply Chains Advancements in Drug Discovery and Development Expanding Frontiers of Artificial Intelligence Ethical Considerations in AI
Tips for Parents to Support and Encourage Kids’ Exploration of Artificial Intelligence
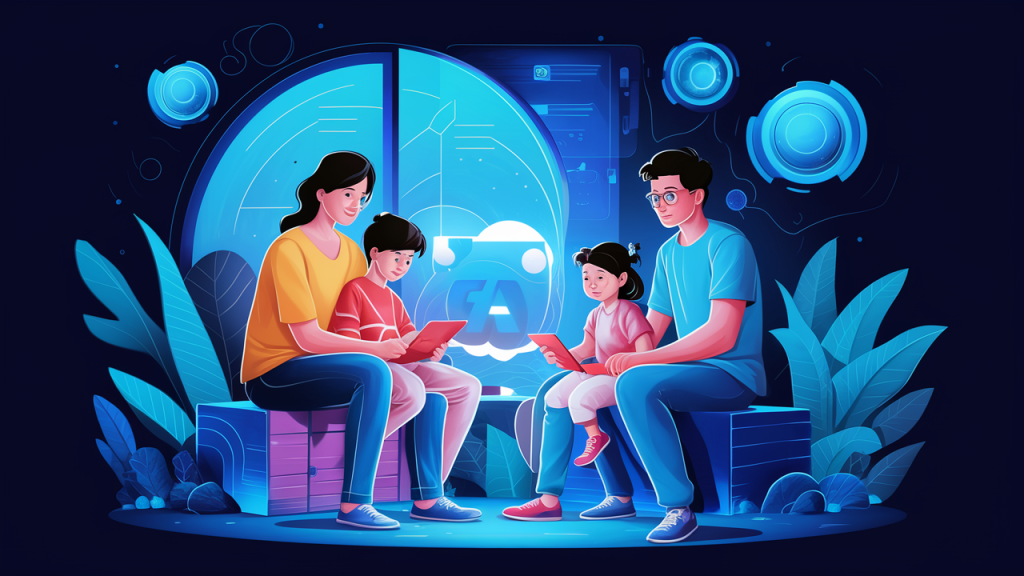
In today’s rapidly evolving digital age, AI for kids is no longer a futuristic concept but a reality that is shaping our world in profound ways. As parents, it’s essential to recognize the immense potential and opportunities that generative AI presents for our children’s education and future careers. By fostering an early understanding and appreciation of this transformative technology, we can empower our kids to become active participants in this exciting frontier. What is AI? Artificial Intelligence, or AI, refers to the development of computer systems capable of performing tasks that typically require human intelligence, such as learning, problem-solving, reasoning, and decision-making. AI systems are designed to mimic human cognitive functions and can continuously improve their performance based on the data they are exposed to. Why is AI Important for Kids? AI is rapidly permeating various aspects of our lives, from virtual assistants and smart home devices to advanced healthcare and transportation systems. By introducing AI concepts to children at an early age, we equip them with the knowledge and skills necessary to navigate and thrive in an AI-driven world. Additionally, exploring generative AI can spark their curiosity, foster critical thinking, and encourage them to approach problems from innovative perspectives. 5 Tips for Parents to Support Children’s AI Exploration 1. Coding and Programming: Introducing kids to coding and programming serves as an excellent gateway to understanding AI. It lays a strong foundation for grasping AI concepts by familiarizing them with the logic and structures underlying computer systems. Platforms like Scratch, Code.org, and Tynker offer engaging, kid-friendly environments where children can learn coding through interactive games and projects. These platforms gamify the learning experience, making it enjoyable and accessible for young learners while simultaneously building their computational thinking and problem-solving skills. 2. Robotics and Electronics: Exploring robotics and electronics provides children with hands-on experience in applying AI concepts to real-world scenarios. Building and programming robots or electronic devices allow them to understand how AI algorithms interact with sensors, actuators, and decision-making processes. Robotics kits such as LEGO Mindstorms or Ozobot offer tangible learning experiences where children can design, build, and program their robots, fostering creativity and critical thinking. Through these activities, kids develop a deeper understanding of AI principles and gain practical skills in engineering and problem-solving. 3. AI-Powered Apps and Games: AI-powered apps and games offer interactive platforms for children to explore AI concepts in a playful and engaging manner. These apps introduce kids to various AI technologies such as machine learning, natural language processing, and computer vision through hands-on activities. For instance, chatbots simulate conversations, language translators facilitate communication across languages, and image recognition apps allow children to interact with AI algorithms in real-time. By interacting with these applications, kids not only learn about AI but also develop digital literacy skills and an understanding of how AI impacts their daily lives. 4. Online Courses and Tutorials: With the growing popularity of generative AI, many online platforms offer age-appropriate courses and tutorials specifically designed for kids. These resources can cover topics ranging from basic AI principles to more advanced concepts like neural networks and deep learning. One such platform is {igebra.ai}, which provides a comprehensive Generative AI Course tailored for young learners. Through interactive lessons and engaging activities, {igebra.ai} introduces children to the fundamentals of generative AI, guiding them from the basics to more complex concepts like creating their own neural networks. This course empowers kids to explore the exciting world of AI in a fun and accessible way, nurturing their curiosity and sparking their interest in this transformative technology. 5. AI-Themed Books and Videos: Books and educational videos can be excellent resources for sparking interest and curiosity about AI. Look for age-appropriate materials that present AI concepts in an engaging and relatable manner, using storytelling or real-world examples. Creating an AI-Friendly Environment at Home To foster a supportive environment for your child’s AI exploration, consider the following tips: Set up a Dedicated Space Create a designated area or corner in your home where your child can work on AI projects, experiment with coding or robotics kits, and engage in hands-on learning activities. 1. Encourage Exploration and Curiosity: Celebrate your child’s curiosity and encourage them to ask questions, explore new ideas, and experiment with different generative AI tools and concepts. Provide them with the resources and support they need to fuel their interests. 2. Collaborate and Learn Together: Don’t be afraid to learn alongside your child. Engage in AI-related activities together, research topics of mutual interest, and collaborate on projects. This shared learning experience can strengthen your bond and deepen your understanding of AI. 3. Connect with Like-Minded Families and Communities: Seek out local or online communities of parents and children interested in AI. Participating in workshops, meetups, or online forums can provide valuable networking opportunities, access to resources, and a support system for your child’s AI journey. The Benefits of Encouraging AI Exploration for Kids 1. Future Career Readiness: AI for kids is reshaping industries and creating new job opportunities that didn’t exist before. By exposing children to AI early on, we prepare them for careers in fields such as robotics, data science, machine learning, and technology development. They will gain a competitive edge and be better equipped to adapt to the ever-changing job market. 2. Enhancing Problem-Solving and Creativity: AI encourages children to think critically, analyze data, and develop creative solutions to complex problems. As they engage with generative AI concepts and tools, they learn to break down challenges into smaller components, identify patterns, and explore alternative approaches to problem-solving. 3. Fostering Collaboration and Communication: Many AI projects involve teamwork and collaboration, which helps children develop essential soft skills like communication, negotiation, and conflict resolution. By working together on AI-related tasks, they learn to appreciate diverse perspectives and leverage each other’s strengths. Final Thought Encouraging your child’s exploration of generative AI is an investment in their future success and a gateway to countless opportunities. By fostering an early understanding and appreciation of this transformative technology, you empower your
What Happens if We Don’t Teach the Application of Generative AI to Our Children?
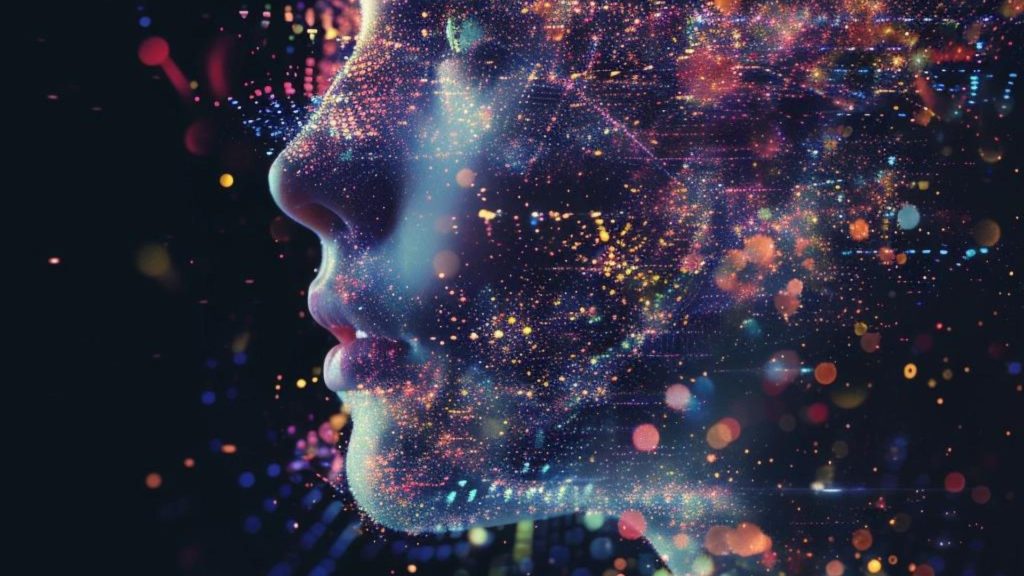
Teaching children about algorithms and data in generative AI is crucial for fostering digital literacy and computational thinking skills from an early age. By introducing concepts like algorithms, data structures, and pattern recognition, children can develop a deeper understanding of how generative AI works and its impact on society. This knowledge empowers them to critically evaluate technology, make informed decisions, and participate responsibly in a data-driven world. Children can grasp the concepts of generative AI through engaging real-world examples tailored to their interests and experiences. For instance, interactive storytelling applications use generative models to create personalized narratives based on user input, sparking children’s imagination and creativity. Similarly, educational games leverage generative AI to adapt content dynamically, providing personalized learning experiences. By experiencing these applications firsthand, children can explore the capabilities of generative AI in a fun and accessible manner, fostering curiosity and excitement about technology. Missed Future Career Opportunities Generative AI is a burgeoning field with vast potential for creativity, problem-solving, and innovation. By not teaching it to kids, we might miss out on opportunities to develop their skills in programming, computational thinking, and creativity. a. Career Paths in AI and Machine Learning for Children Interested in Technology As children develop an interest in technology, exploring career paths in AI and machine learning opens up a world of possibilities. They can pursue roles such as data scientist, machine learning engineer, or AI researcher, where they apply algorithms and data to solve complex problems across various domains. Additionally, careers in AI ethics and policy offer opportunities to shape the ethical and responsible use of AI technology. By acquiring foundational skills in mathematics, programming, and critical thinking, children can embark on rewarding careers that drive innovation and positive societal impact in the rapidly evolving field of AI. b. The Role of Generative AI in Emerging Industries like Virtual Reality, Augmented Reality, and Robotics Generative AI plays a pivotal role in shaping the future of emerging industries such as virtual reality (VR), augmented reality (AR), and robotics. In VR and AR, generative models enable realistic simulation and rendering of virtual environments, enhancing immersion and user experience. Similarly, in robotics, generative AI algorithms facilitate tasks like motion planning, object manipulation, and human-robot interaction. By leveraging generative AI techniques, children interested in these fields can contribute to groundbreaking advancements that redefine how we interact with technology and perceive the world around us. c. Entrepreneurial Opportunities for Children with Skills in Generative AI For children with skills in generative AI, entrepreneurial opportunities abound in various sectors. They can create innovative products and services leveraging generative AI, such as custom content generation platforms, personalized recommendation systems, or AI-driven creative tools. Moreover, they can establish startups focused on niche areas like generative art, music composition, or fashion design, disrupting traditional industries with AI-powered solutions. By combining their technical expertise with entrepreneurial vision, children can embark on exciting ventures that drive economic growth and societal transformation in the digital age. Lack of Innovation and Creativity Generative AI has the potential to drive innovation across various industries, from art and design to healthcare and finance. By not educating kids about this technology, we may stifle their ability to explore new possibilities and create innovative solutions to complex problems. a. How Generative AI Can Inspire Storytelling and Narrative Creation? Generative AI offers storytellers and writers a powerful tool for sparking creativity and exploring new narrative possibilities. By leveraging natural language processing (NLP) models like GPT (Generative Pre-trained Transformer), authors can collaborate with AI to generate plot ideas, character development arcs, and even entire storylines on tools such as ChatGPT. This collaborative approach encourages experimentation and pushes the boundaries of traditional storytelling conventions, leading to the creation of immersive and engaging narratives that captivate audiences across diverse genres and mediums. b. Using AI for Generating Music, Art, and Literature: Encouraging Creative Exploration Generative AI has revolutionized the fields of music composition, visual art, and literary creation by enabling artists to explore new creative avenues and push the limits of their imagination. AI algorithms, such as deep neural networks and recurrent neural networks, can analyze vast amounts of existing data and learn to generate original music compositions, visual artworks, and literary works that resonate with human emotions and aesthetics. Midjourney as a versatile tool designs logos, crafts illustrations, and generates memes. Other tools such as Stableaudio enables pro users to craft music for commercial projects and basic tier users for non-commercial ones, enhancing creativity. This democratization of creativity empowers artists of all backgrounds to experiment with AI-driven tools, unleashing a wave of innovation and artistic expression across diverse cultural landscapes. c. Collaborative AI Projects: Fostering Teamwork and Problem-Solving Skills Collaborative AI projects provide an excellent opportunity for individuals to develop teamwork and problem-solving skills while tackling complex challenges in interdisciplinary teams. By working together to design, implement, and deploy AI systems, participants learn to leverage their unique strengths and expertise to achieve common goals effectively. Moreover, collaborative AI projects foster communication, collaboration, and leadership skills essential for success in the rapidly evolving field of AI and beyond. Whether developing AI-powered applications, conducting research experiments, or participating in hackathons, collaborative projects cultivate a culture of innovation and collaboration that drives meaningful societal impact. Increased the Digital Divide If we neglect teaching generative AI applications to children, it exacerbates the digital divide. Those without access or understanding of these technologies will struggle to compete in a digitally-driven world, widening the gap between those who can harness AI’s potential and those left behind, perpetuating inequality and limiting opportunities. a. Bridging the Gap: Providing Access to AI Education in Underserved Communities Bridging the digital divide requires proactive efforts to ensure that AI education is accessible to all, regardless of socioeconomic status or geographic location. Initiatives such as community-based AI workshops, online learning platforms, and outreach programs in underserved areas can empower individuals with the knowledge and skills needed to thrive in the digital age. By collaborating with local schools, libraries, and community centers, organizations can create inclusive
Why Should Schools Take the Lead in Using and Teaching Generative AI?
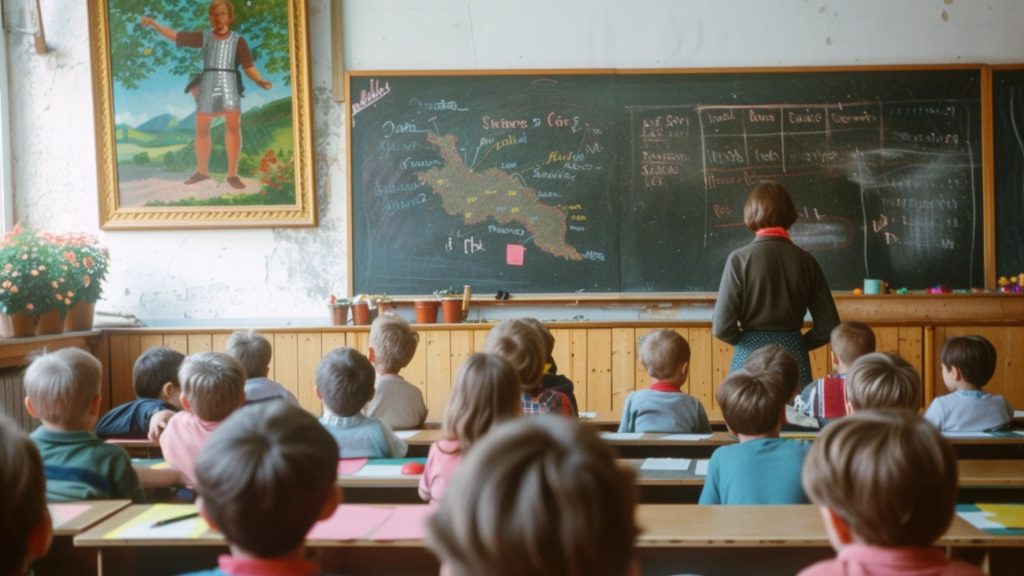
Generative AI focuses on creating new data or content rather than simply analyzing existing information or making predictions based on patterns. Unlike traditional AI, which primarily relies on predefined rules and structured data, Generative AI systems have the ability to generate novel outputs, such as images, text, or audio, that mimic human creativity and intelligence. The importance of AI in education cannot be overstated. As the world becomes increasingly driven by technology, it is essential for educational institutions to equip students with the skills and knowledge they need to thrive in the digital age. AI has the power to enhance teaching effectiveness, improve learning outcomes, and prepare students for the jobs of the future. By embracing AI in education, we can empower children to become lifelong learners who are adaptable, critical thinkers, and well-prepared to thrive in an ever-evolving digital world. Benefits of Integrating Generative AI in Education Through Schools The integration of Generative AI holds immense promise for transforming education by offering a myriad of potential benefits: a. Personalized Learning Experiences Generative AI enables the creation of personalized learning materials tailored to individual student needs and preferences. By analyzing student data and learning patterns, AI algorithms can generate adaptive content, exercises, and tutorials, facilitating personalized learning journeys that cater to diverse learning styles and abilities. b. Enhanced Creativity and Innovation Generative AI tools empower students and educators to unleash their creativity and explore new frontiers of innovation. From generating artwork and music compositions to crafting storytelling narratives, AI-driven creativity tools inspire experimentation and collaboration, fostering a culture of innovation and expression in educational settings. c. Automated Assessment and Feedback AI-powered assessment tools streamline the process of evaluating student performance and providing timely feedback. Through machine learning algorithms, educators can automate grading tasks, analyze student responses, and offer personalized feedback, freeing up valuable time for more meaningful interactions and instructional activities. d. Real-world Application and Problem Solving Generative AI equips students with practical skills and problem-solving abilities relevant to real-world challenges. By engaging with AI-driven simulations, virtual laboratories, and scenario-based learning experiences, students gain hands-on experience in applying theoretical concepts to real-world scenarios, preparing them for future careers and societal challenges. Potential GenAI Applications at Schools Here are some compelling examples of potential GenAI applications that can be used at schools. a. AI-based Tutoring Systems AI-based tutoring systems utilize sophisticated algorithms to analyze individual learning patterns and provide tailored feedback and guidance. Many platforms leverage AI to offer interactive lessons and adaptive exercises in subjects ranging from mathematics to language learning. By adapting content and pacing to match each student’s proficiency level, AI tutoring systems support self-paced learning and help students achieve mastery in diverse subject areas. Moreover, they offer immediate feedback and targeted interventions, empowering students to address their learning gaps and maximize their academic potential. b. Smart Classrooms and Learning Management Systems Smart classrooms integrate technology such as interactive whiteboards, tablets, and smart projectors to create interactive and engaging learning environments. LMS platforms utilize AI algorithms to streamline administrative tasks, deliver personalized learning content, and track student progress. These systems facilitate seamless communication between teachers and students, enable collaborative learning activities, and provide access to a wealth of educational resources. c. Virtual Reality (VR) and Augmented Reality (AR) Applications In education, VR and AR offer innovative ways to engage students and enhance understanding of complex concepts. For example, students can virtually explore historical sites, dissect virtual organisms, or conduct science experiments in simulated environments. AR applications can overlay digital annotations onto physical objects, making learning materials more interactive and engaging. Additionally, VR and AR enable remote collaboration and virtual field trips, breaking down geographical barriers and providing access to educational experiences that may not be possible in traditional classrooms. d. Assistive Technologies for Special Education Assistive technologies aim to enhance accessibility, facilitate communication, and promote independence in educational settings. One example of assistive technology is text-to-speech software, which converts written text into spoken language, enabling students with visual impairments or reading difficulties to access written materials more easily. Similarly, speech recognition software allows students with motor disabilities to input text using voice commands, enhancing their ability to participate in writing activities. Other examples of assistive technologies include communication devices such as augmentative and alternative communication (AAC) systems, which help nonverbal students communicate effectively using symbols, pictures, or speech-generating devices. For students with learning disabilities, assistive technologies like graphic organizers and mind-mapping software can support organization, comprehension, and information processing. Moreover, specialized hardware such as adaptive keyboards, switches, and joysticks enable students with physical disabilities to navigate digital interfaces and interact with computers more effectively. Challenges in Implementing Generative AI in Schools a. Despite the potential benefits, implementing Generative AI in educational settings comes with its own set of challenges. One significant hurdle is the lack of resources and infrastructure necessary to support AI integration. Many schools may not have access to the computational power or expertise required to deploy and maintain AI systems effectively. b. Resistance to technological change is another obstacle that schools may encounter. Educators and administrators may be hesitant to adopt new technologies due to concerns about their effectiveness, relevance to the curriculum, or impact on traditional teaching methods. Overcoming this resistance requires proactive efforts to demonstrate the value of Generative AI and provide support and training to educators. c. Security and privacy concerns also loom large in the implementation of Generative AI in schools. Given the sensitive nature of student data, educators must ensure that AI systems are deployed in compliance with relevant regulations and guidelines. There is a need for robust data protection measures and transparent policies to address potential risks such as data breaches or algorithmic biases. Ethical Considerations and Concerns In the realm of integrating Generative AI into educational settings, ethical considerations play a pivotal role in shaping its implementation. Four primary ethical concerns arise: a. Bias and Fairness Issues In educational contexts, biased algorithms can perpetuate existing inequalities by favoring certain demographic groups or reinforcing stereotypes. It’s crucial to
How Healthcare Industry is Using Generative AI?
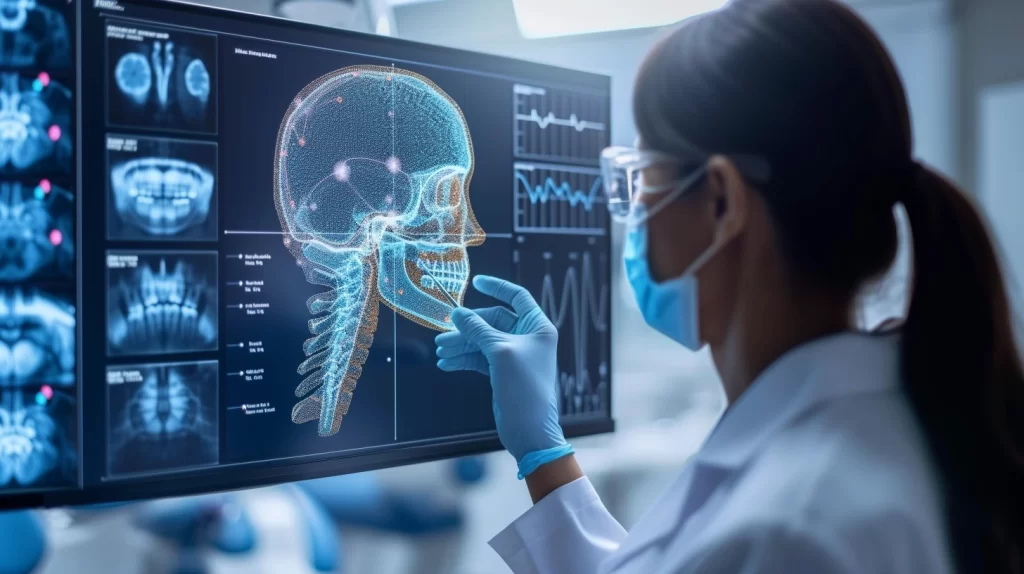
According to Statista, Healthcare holds a dominant position in the adoption of AI technologies, with nearly 16 percent of the AI market dedicated to healthcare applications. Among these AI technologies, Generative AI stands out for its ability to create new data, images, or text, often indistinguishable from human-created content. It operates on principles like Generative Adversarial Networks (GANs) or Variational Autoencoders (VAEs), enabling machines to learn and replicate patterns from existing data to create entirely new outputs. The healthcare industry has rapidly embraced AI technologies to enhance patient care, streamline administrative processes, and advance medical research. From AI-powered diagnostic tools to predictive analytics for patient outcomes, these technologies have proven instrumental in improving efficiency, accuracy, and overall quality of care in healthcare settings. Applications of Generative AI in Healthcare Generative AI in healthcare facilitates medical image generation, aiding in diagnostics and treatment planning. It also assists in drug discovery by generating molecular structures. Additionally, it generates synthetic patient data for training AI models, enhancing privacy and security. Moreover, it supports personalised medicine through the generation of tailored treatment plans based on individual patient data. 1. Medical Image Generation Generative AI in medical image generation synthesizes realistic images for training machine learning models, enhancing diagnostic accuracy and treatment planning. It generates diverse medical images, including X-rays, MRI scans, and CT scans, aiding in disease detection and monitoring. This technology accelerates research and development in medical imaging, improving patient care outcomes. a. Generating Synthetic Medical Images for Training AI Models Generative AI techniques, such as Generative Adversarial Networks (GANs) and Variational Autoencoders (VAEs), play a pivotal role in generating synthetic medical images. These images are invaluable for training AI models, providing a diverse and extensive dataset that encompasses various medical conditions, anatomical variations, and imaging modalities. By synthesizing images, Generative AI mitigates the challenges associated with limited or inaccessible real-world data, facilitating robust training and enhancing the generalization capabilities of AI algorithms. Case Study : Medical Image Generation b. Improving Diagnostic Accuracy and Speed The utilization of synthetic medical images generated by Generative AI contributes significantly to enhancing diagnostic accuracy and expediting the interpretation process. AI models trained on a comprehensive dataset of synthesized images demonstrate superior performance in identifying subtle abnormalities, distinguishing between similar pathologies, and accurately diagnosing complex medical conditions. Furthermore, the availability of a larger and more diverse training dataset enables AI algorithms to refine their learning, resulting in more precise and reliable diagnoses. 2. Drug Discovery and Development Generative AI accelerates drug discovery by generating novel molecular structures with desired properties, expediting the identification of potential drug candidates. It facilitates the design of new compounds with optimized pharmacological profiles, reducing the time and cost of drug development. This technology holds promise for addressing unmet medical needs and advancing pharmaceutical innovation. a. Generating Molecular Structures for Drug Candidates Generative AI is revolutionizing drug discovery by generating molecular structures for potential drug candidates. Using algorithms such as recurrent neural networks (RNNs) and reinforcement learning, Generative AI designs novel molecules with desired pharmacological properties. These generated structures provide valuable insights into chemical space exploration, enabling researchers to identify promising drug candidates that exhibit high potency, specificity, and safety profiles. Case Study : Drug Discovery b. Accelerating the Drug Discovery Process Traditional methods of drug development are time-consuming and labor-intensive, often requiring years of experimentation and testing. However, Generative AI expedites this process by rapidly generating and screening vast libraries of molecular structures. Through virtual screening and computational analysis, researchers can prioritise lead compounds for further experimental validation, reducing the time and resources required to bring new drugs to market. 3. Personalised Medicine Generative AI tailors medical interventions to individuals by analyzing their unique health data and generating personalized treatment plans. It predicts patient responses to therapies, accounting for genetic, environmental, and lifestyle factors. This approach enhances treatment efficacy and safety, optimising healthcare outcomes and improving patient satisfaction. a. Generating Patient-specific Treatment Plans By analyzing vast datasets of patient information, including genomic data, medical history, and treatment outcomes, Generative AI algorithms develop personalized therapeutic strategies that optimize efficacy while minimizing adverse effects. These tailored treatment plans ensure that patients receive the most effective interventions based on their specific healthcare needs and genetic predispositions. b. Enhancing Patient Outcomes and Reducing Side Effects By accounting for individual variability in drug response and susceptibility to side effects, personalized treatment plans optimize therapeutic efficacy while minimizing the risk of adverse events. This precision medicine approach not only enhances patient satisfaction and adherence to treatment but also leads to better clinical outcomes, reduced hospitalizations, and improved quality of life for patients. Case Study : Personalized Medicine 4. Natural Language Processing (NLP) in Healthcare Natural Language Processing (NLP) in healthcare automates medical documentation, extracting valuable insights from clinical notes, electronic health records (EHRs), and research literature. It aids in patient management, clinical decision support, and population health management. NLP also enhances healthcare communication, enabling efficient information exchange between patients, providers, and researchers. a. Generating Medical Reports and Summaries NLP algorithms analyze clinical notes, diagnostic imaging reports, and electronic health records (EHRs) to extract relevant information and generate comprehensive patient summaries. This automation streamlines the documentation process, improves the accuracy and completeness of medical records, and frees up healthcare professionals to focus on patient care. b. Supporting the Clinical Decision-making Process By analyzing textual data from medical journals, textbooks, and clinical trials, NLP algorithms provide healthcare providers with evidence-based insights, treatment recommendations, and differential diagnoses. This real-time decision support enhances diagnostic accuracy, facilitates evidence-based practice, and ultimately improves patient outcomes. Challenges and Ethical Considerations a. Data Privacy and Security Concerns The proliferation of Generative AI in healthcare raises significant concerns regarding the privacy and security of sensitive patient data. As AI algorithms become increasingly sophisticated, there is a heightened risk of data breaches and unauthorized access to confidential medical information. Addressing these concerns requires robust data encryption, access controls, and compliance with stringent data protection regulations such as HIPAA. b. Bias and Fairness in AI-generated Outputs Another challenge
The Power of Math and AI: Synergies for the Future
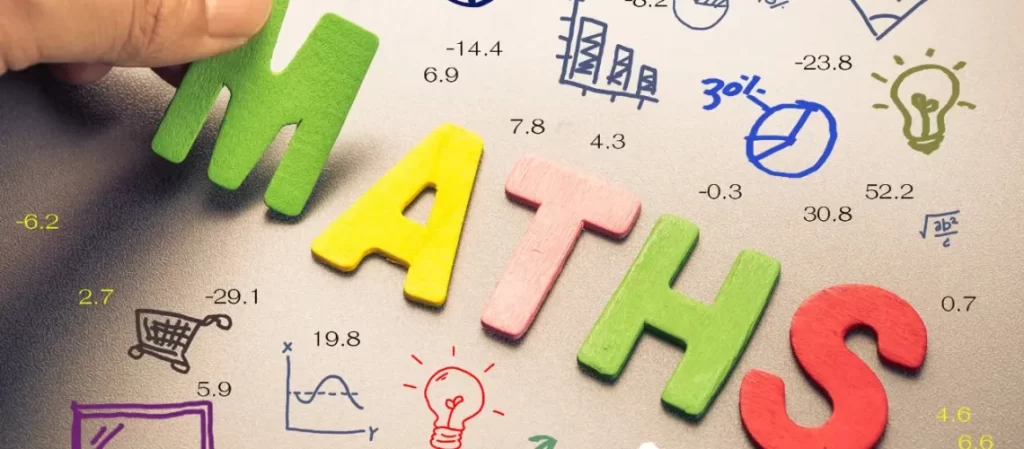
The value of maths and AI in the quickly evolving world of today cannot be emphasized. Both math and AI play integral roles in various aspects of our lives, from scientific research and technological advancements to everyday decision-making processes. Let’s delve into the significance of each discipline individually. Mathematics, as a fundamental discipline, provides a language and framework for understanding and describing the world around us. It offers precise methods for calculation, measurement, and logical reasoning. We can create representations of complex systems through mathematical modeling and simulation, enabling us to study and predict their behavior. Mathematical models help us make informed decisions in diverse fields, including physics, engineering, economics, and even social sciences. Moreover, math plays a pivotal role in predictive analytics and forecasting. We can make educated predictions about future trends and outcomes by analyzing historical data and applying mathematical techniques, such as regression and time series analysis. These predictions are invaluable for businesses, financial institutions, and even weather forecasting agencies, aiding in strategic planning, risk management, and resource allocation. The Role of Mathematics in AI Development a. Mathematical Foundations of AI Algorithms 1. Probability and Statistics in Machine Learning – Probability theory and statistical inference – Bayesian methods and probabilistic graphical models – Estimation, hypothesis testing, and model selection 2. Linear Algebra in Neural Networks – Matrix operations and transformations – Vector spaces and linear transformations – Eigenvalues and eigenvectors for dimensionality reduction 3. Calculus in Optimisation Algorithms – Gradient-based optimisation methods – Optimization of loss functions in training algorithms – Differential equations in dynamic systems modelling b. Mathematical Modelling for AI Applications 1. Data Analysis and Pattern Recognition – Methods for identifying statistical patterns – Feature extraction and dimensionality reduction – Clustering and classification algorithms 2. Predictive Modeling and Forecasting – Forecasting and time series analysis techniques – Predictive analytics and regression models – Markov chains and hidden Markov models 3. Optimization and Decision-Making – Convex optimization for confined situations – Linear and nonlinear programming – Using game theory and decision theory to make the best decisions possible AI Advancements Enhancing Mathematics a. AI-driven Mathematical Discovery AI-driven mathematical discovery involves two key aspects: automated theorem proving and the discovery of new mathematical concepts. 1. Automated Theorem Proving Automated theorem proving utilizes AI algorithms to prove mathematical theorems. These algorithms employ automated deduction systems and formal logic to derive conclusions from axioms and inference rules. By automating the proof process, AI systems can efficiently explore vast mathematical spaces and generate rigorous mathematical proofs. This has the potential to significantly accelerate mathematical research and enable the verification of complex theorems that would otherwise be challenging for humans to prove manually. 2. Discovery of New Mathematical Concepts The discovery of new mathematical concepts through AI algorithms represents an exciting frontier in mathematics. By harnessing the power of machine learning and deep learning techniques, AI can assist in exploring uncharted mathematical territories and identifying novel concepts that may have eluded human mathematicians. One approach to discovering new mathematical concepts involves training neural networks on large datasets of mathematical structures, formulas, and patterns. These networks can learn to recognize regularities and correlations within the data and generate new mathematical expressions or structures based on the learned patterns. This process is similar to how neural networks are used in fields like computer vision or natural language processing to generate novel images or texts. b. AI-assisted Problem Solving 1. Optimization and Constraint Satisfaction AI-assisted problem-solving in optimization and constraint satisfaction involves utilizing AI algorithms to tackle complex optimization problems and find satisfactory solutions within given constraints. Finding the best or optimal answer from a list of potential solutions is a task that can be accomplished with the help of AI algorithms. These problems often arise in various domains, such as logistics, scheduling, resource allocation, and production planning. 2. Mathematical Conjecture Testing Mathematical conjecture testing involves using AI techniques to verify or falsify mathematical conjectures, which are statements that have not been proven or disproven yet. AI algorithms can play a significant role in accelerating the process of testing and validating conjectures, offering computational power and exploration capabilities that complement human mathematical intuition. c. AI-Enabled Mathematical Education 1. Personalized Learning and Adaptive Instructio To design individualized learning routes, AI algorithms can examine students’ learning styles, aptitudes, and deficiencies. By collecting and analyzing data on student’s performance, preferences, and progress, AI systems can adapt instructional content and techniques to match individual needs. With the aid of this individualized approach, students can learn at their own pace, receive specialized instruction, and concentrate on areas that require improvement. 2. Intelligent Tutoring Systems Intelligent tutoring systems (ITS) driven by AI offer pupils customized direction and support throughout their arithmetic learning process. These systems communicate with students, comprehend their questions, and offer personalized explanations or solutions using natural language processing and machine learning techniques. ITS can simulate one-on-one tutoring sessions, identify misconceptions, offer step-by-step guidance, and adapt instructional strategies based on individual needs. 3. Automated Assessment and Feedback AI algorithms can automate the process of assessing mathematical assignments, quizzes, or exams. By employing techniques such as machine learning, pattern recognition, and natural language processing, AI systems can evaluate student responses, identify errors or misconceptions, and provide timely feedback. Automated assessment saves time for educators, allows for immediate feedback to students, and offers consistent grading standards. Synergistic Applications of Math and AI a. Data-driven Science and Research 1. Computational Biology and Genomics The field of computational biology and genomics heavily relies on data-driven approaches to analyze vast amounts of biological data. With the advent of high-throughput sequencing technologies, large-scale genomic datasets have become available, enabling researchers to uncover patterns, identify genetic variations, and understand the mechanisms underlying complex biological processes. 2. Climate Modelling and Environmental Sciences Data-driven approaches play a crucial role in climate modeling and environmental sciences, where large-scale datasets from various sources, such as satellites, weather stations, and climate models, are analyzed to understand and predict climate patterns and environmental changes. 3. High-Energy Physics and Cosmology High-energy