According to Statista, Healthcare holds a dominant position in the adoption of AI technologies, with nearly 16 percent of the AI market dedicated to healthcare applications. Among these AI technologies, Generative AI stands out for its ability to create new data, images, or text, often indistinguishable from human-created content. It operates on principles like Generative Adversarial Networks (GANs) or Variational Autoencoders (VAEs), enabling machines to learn and replicate patterns from existing data to create entirely new outputs.
The healthcare industry has rapidly embraced AI technologies to enhance patient care, streamline administrative processes, and advance medical research. From AI-powered diagnostic tools to predictive analytics for patient outcomes, these technologies have proven instrumental in improving efficiency, accuracy, and overall quality of care in healthcare settings.
Applications of Generative AI in Healthcare
Generative AI in healthcare facilitates medical image generation, aiding in diagnostics and treatment planning. It also assists in drug discovery by generating molecular structures. Additionally, it generates synthetic patient data for training AI models, enhancing privacy and security. Moreover, it supports personalised medicine through the generation of tailored treatment plans based on individual patient data.
1. Medical Image Generation
Generative AI in medical image generation synthesizes realistic images for training machine learning models, enhancing diagnostic accuracy and treatment planning. It generates diverse medical images, including X-rays, MRI scans, and CT scans, aiding in disease detection and monitoring. This technology accelerates research and development in medical imaging, improving patient care outcomes.
a. Generating Synthetic Medical Images for Training AI Models
Generative AI techniques, such as Generative Adversarial Networks (GANs) and Variational Autoencoders (VAEs), play a pivotal role in generating synthetic medical images. These images are invaluable for training AI models, providing a diverse and extensive dataset that encompasses various medical conditions, anatomical variations, and imaging modalities. By synthesizing images, Generative AI mitigates the challenges associated with limited or inaccessible real-world data, facilitating robust training and enhancing the generalization capabilities of AI algorithms.
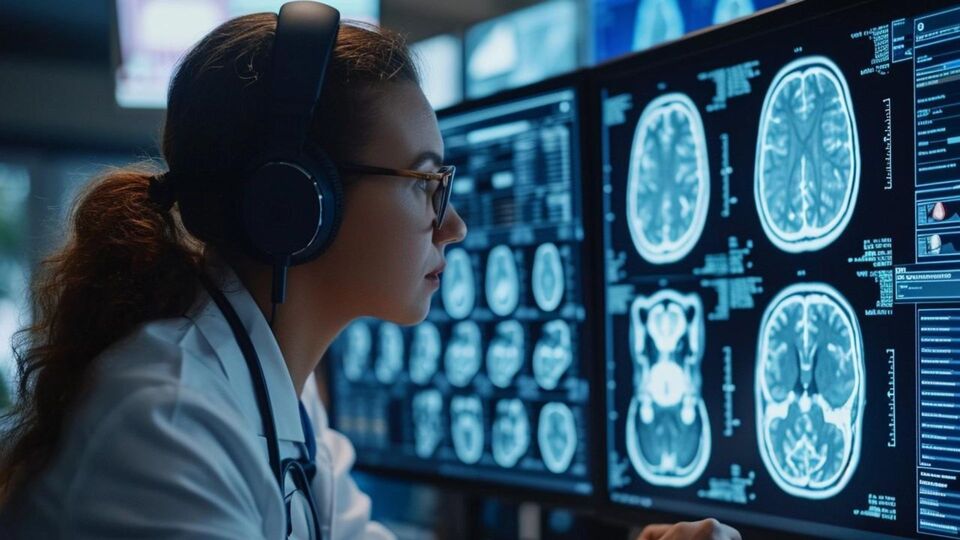
Case Study : Medical Image Generation
- University of Michigan: The University of Michigan has developed a generative AI model for simulating scenarios related to sepsis, improving medical training and simulation within healthcare settings.
- University of Pennsylvania: Researchers at the University of Pennsylvania have deployed a generative AI model to simulate the spread of COVID-19, enabling them to evaluate the impact of interventions like social distancing and vaccination on the virus.
- Google: Google developed an LLM for medical, Med-PaLM 2, which achieved over 85% accuracy in responding to US Medical Licensing Examination queries. This AI solution aims to provide precise responses to medical inquiries, enhancing diagnostic capabilities.
- Research Studies: Researchers have experimented with Generative Adversarial Network (GAN) models to extract and enhance features in medical images like retinal images and ultrasound scans, demonstrating improved image quality compared to traditional methods.
b. Improving Diagnostic Accuracy and Speed
The utilization of synthetic medical images generated by Generative AI contributes significantly to enhancing diagnostic accuracy and expediting the interpretation process. AI models trained on a comprehensive dataset of synthesized images demonstrate superior performance in identifying subtle abnormalities, distinguishing between similar pathologies, and accurately diagnosing complex medical conditions. Furthermore, the availability of a larger and more diverse training dataset enables AI algorithms to refine their learning, resulting in more precise and reliable diagnoses.
2. Drug Discovery and Development
Generative AI accelerates drug discovery by generating novel molecular structures with desired properties, expediting the identification of potential drug candidates. It facilitates the design of new compounds with optimized pharmacological profiles, reducing the time and cost of drug development. This technology holds promise for addressing unmet medical needs and advancing pharmaceutical innovation.
a. Generating Molecular Structures for Drug Candidates
Generative AI is revolutionizing drug discovery by generating molecular structures for potential drug candidates. Using algorithms such as recurrent neural networks (RNNs) and reinforcement learning, Generative AI designs novel molecules with desired pharmacological properties. These generated structures provide valuable insights into chemical space exploration, enabling researchers to identify promising drug candidates that exhibit high potency, specificity, and safety profiles.
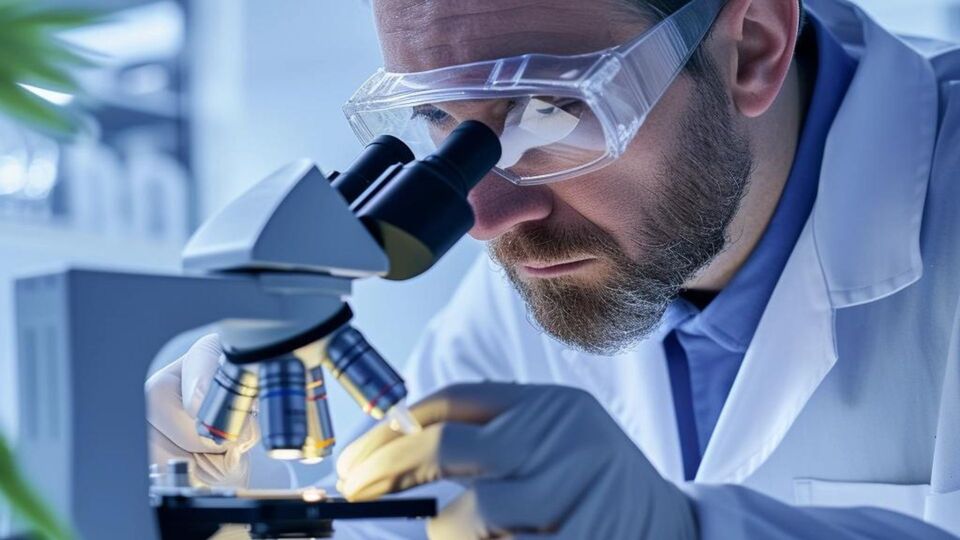
Case Study : Drug Discovery
- Insilico Medicine: Insilico Medicine, a pioneer in generative AI for drug discovery, has developed a generative adversarial network-based AI platform that accelerates the generation of novel molecules, disease target identification, and prediction of clinical trial outcomes. This platform led to the discovery and design of the world’s first AI-generated anti-fibrotic small molecule inhibitor (INS018_055), which has progressed through Phase 0 and Phase I trials and entered Phase II clinical trials.
- Adaptyv Bio: Switzerland-based biotechnology startup Adaptyv Bio created a platform utilizing generative AI for protein engineering. Their platform integrates generative algorithms, advanced robotics, microfluidics, and synthetic biology techniques to generate and optimize protein sequences, enhancing protein engineering processes.
- Iktos and Curreio Partnership: Iktos partnered with Curreio, a Japanese cryo-electron microscopy expert, to leverage AI and cryo-EM technology for drug discovery. Iktos’s generative AI technology, Makya, benefits from insights gained through cryo-EM analysis to design molecular structures that optimize protein-ligand interactions, accelerating the drug discovery process.
- NVIDIA BioNeMo: NVIDIA’s BioNeMo platform offers services to develop, customize, and deploy foundation models for drug discovery. Pharmaceutical companies and tech bios utilize BioNeMo to develop generative AI models for protein structure prediction, molecular optimization, docking prediction, and more. This platform enables the integration of generative AI to reduce experiments and enhance drug research and development processes.
b. Accelerating the Drug Discovery Process
Traditional methods of drug development are time-consuming and labor-intensive, often requiring years of experimentation and testing. However, Generative AI expedites this process by rapidly generating and screening vast libraries of molecular structures. Through virtual screening and computational analysis, researchers can prioritise lead compounds for further experimental validation, reducing the time and resources required to bring new drugs to market.
3. Personalised Medicine
Generative AI tailors medical interventions to individuals by analyzing their unique health data and generating personalized treatment plans. It predicts patient responses to therapies, accounting for genetic, environmental, and lifestyle factors. This approach enhances treatment efficacy and safety, optimising healthcare outcomes and improving patient satisfaction.
a. Generating Patient-specific Treatment Plans
By analyzing vast datasets of patient information, including genomic data, medical history, and treatment outcomes, Generative AI algorithms develop personalized therapeutic strategies that optimize efficacy while minimizing adverse effects. These tailored treatment plans ensure that patients receive the most effective interventions based on their specific healthcare needs and genetic predispositions.
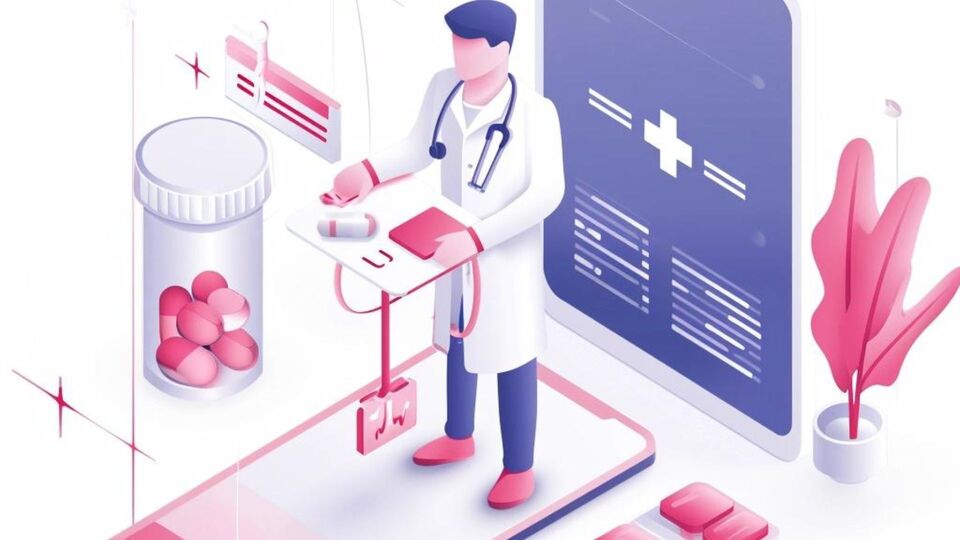
b. Enhancing Patient Outcomes and Reducing Side Effects
By accounting for individual variability in drug response and susceptibility to side effects, personalized treatment plans optimize therapeutic efficacy while minimizing the risk of adverse events. This precision medicine approach not only enhances patient satisfaction and adherence to treatment but also leads to better clinical outcomes, reduced hospitalizations, and improved quality of life for patients.
Case Study : Personalized Medicine
- Insilico Medicine: Insilico Medicine has developed a generative adversarial network-based AI platform that accelerates the generation of novel molecules, disease target identification, and prediction of clinical trial outcomes. This platform led to the discovery and design of the world’s first AI-generated anti-fibrotic small molecule inhibitor (INS018_055), which has progressed through clinical trials, showcasing the potential of generative AI in personalized medicine.
- University of Michigan: The University of Michigan has implemented a generative AI model for simulating scenarios related to sepsis, enhancing medical training and simulation within healthcare settings. This application of generative AI contributes to personalized training experiences for healthcare professionals, improving their preparedness and response to critical medical situations.
- Iktos and Curreio Partnership: Iktos collaborated with Curreio to leverage generative AI and cryo-electron microscopy technology for drug discovery. By integrating insights from cryo-EM analysis into generative AI technology, they designed molecular structures optimizing protein-ligand interactions, showcasing how personalized approaches can be enhanced through advanced technologies in drug discovery.
4. Natural Language Processing (NLP) in Healthcare
Natural Language Processing (NLP) in healthcare automates medical documentation, extracting valuable insights from clinical notes, electronic health records (EHRs), and research literature. It aids in patient management, clinical decision support, and population health management. NLP also enhances healthcare communication, enabling efficient information exchange between patients, providers, and researchers.
a. Generating Medical Reports and Summaries
NLP algorithms analyze clinical notes, diagnostic imaging reports, and electronic health records (EHRs) to extract relevant information and generate comprehensive patient summaries. This automation streamlines the documentation process, improves the accuracy and completeness of medical records, and frees up healthcare professionals to focus on patient care.
b. Supporting the Clinical Decision-making Process
By analyzing textual data from medical journals, textbooks, and clinical trials, NLP algorithms provide healthcare providers with evidence-based insights, treatment recommendations, and differential diagnoses. This real-time decision support enhances diagnostic accuracy, facilitates evidence-based practice, and ultimately improves patient outcomes.
Challenges and Ethical Considerations
a. Data Privacy and Security Concerns
The proliferation of Generative AI in healthcare raises significant concerns regarding the privacy and security of sensitive patient data. As AI algorithms become increasingly sophisticated, there is a heightened risk of data breaches and unauthorized access to confidential medical information. Addressing these concerns requires robust data encryption, access controls, and compliance with stringent data protection regulations such as HIPAA.
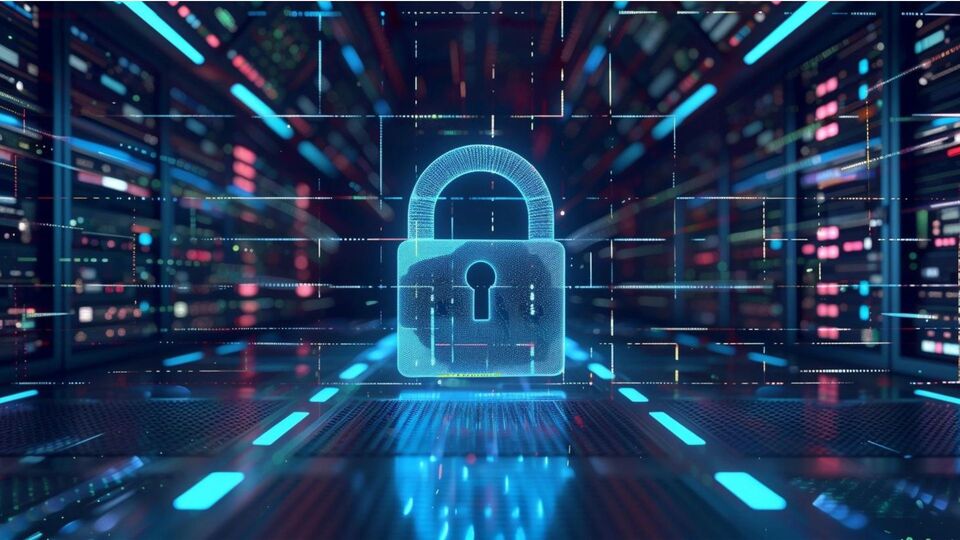
b. Bias and Fairness in AI-generated Outputs
Another challenge is the potential for bias and unfairness in AI-generated outputs, which could perpetuate disparities in healthcare delivery. Biases may arise from imbalances in training data, leading to inaccurate predictions or recommendations, particularly for underrepresented patient populations. Mitigating bias requires comprehensive data curation, algorithmic transparency, and ongoing monitoring to ensure fairness and equity in AI applications.
c. Regulatory Compliance and Standards
The healthcare industry must navigate complex regulatory frameworks and standards when deploying Generative AI technologies. Compliance with regulations such as the FDA’s guidelines for AI-based medical devices and adherence to industry standards for data interoperability and security are paramount. Establishing clear guidelines and best practices for AI development, validation, and deployment is essential to ensure patient safety and regulatory compliance.
d. Ensuring Transparency and Accountability
Maintaining transparency and accountability in AI-driven healthcare is essential to foster trust among patients, healthcare providers, and regulatory agencies. Stakeholders must have access to clear explanations of how AI algorithms make decisions and the potential risks associated with their use. Moreover, mechanisms for auditing and oversight are needed to hold developers and users accountable for the ethical and responsible deployment of AI technologies.
Advantages for Patients and Physicians
The use of Generative AI can reduce waiting times for consultations by streamlining administrative tasks and providing continuous patient monitoring through sensors, leading to more in-depth care. Generative AI can share medical advice and data-driven information with patients, empowering them with better knowledge of their health status and facilitating informed decision-making.
Nearly all healthcare providers and executives believe that advancements in Generative AI could free up to 40% of all working hours through language-based AI support, allowing doctors to focus on higher-value tasks. This technology revolutionises healthcare by generating new medical images like X-rays or MRIs, aiding in accurate diagnostics and personalised treatment regimens based on patients’ medical history.
Future Trends and Opportunities
a. Integration of Generative AI with Other Healthcare Technologies
The integration of Generative AI with other healthcare technologies, such as wearable devices, electronic health records (EHRs), and telemedicine platforms, holds immense potential to enhance patient care and outcomes. By leveraging AI-generated insights to inform clinical decision-making and personalised interventions, healthcare providers can deliver more proactive, efficient, and patient-centered care.
b. Expansion into New Areas such as Genomics and Digital Pathology
Generative AI is poised to revolutionize fields such as genomics and digital pathology, enabling advanced analysis and interpretation of complex biological data. By generating synthetic genomic sequences and histopathological images, AI algorithms can accelerate research in precision medicine, biomarker discovery, and disease diagnosis. This expansion into new areas promises to unlock novel insights into disease mechanisms and therapeutic targets, paving the way for personalized and targeted therapies.
AI and Doctors Shape Healthcare Together
AI has become a transformative force in the healthcare industry, revolutionizing diagnostics, treatment, and patient care. Its ability to analyze vast datasets, identify patterns, and provide insights has led to improved accuracy, efficiency, and personalized medicine. However, amidst these advancements, the central role of doctors cannot be overlooked. While AI enhances healthcare delivery, doctors bring indispensable expertise, empathy, and human judgment to the table. In moments of uncertainty or complexity, it is the collaboration between AI and doctors that ensures comprehensive and effective care, underscoring the importance of human intervention and the irreplaceable value of medical professionals in healthcare.
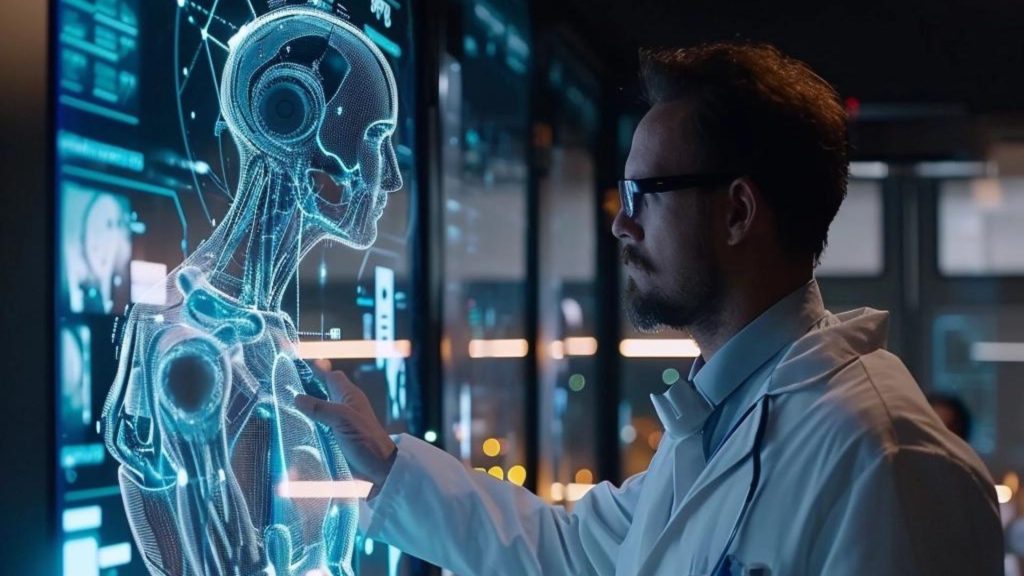
Final Thoughts
As Generative AI continues to reshape the healthcare landscape, there is a pressing need for ongoing research, development, and responsible deployment of AI technologies. By addressing challenges related to data privacy, bias, regulatory compliance, and transparency, stakeholders can harness the transformative potential of AI to improve patient care, enhance clinical outcomes, and advance healthcare innovation.
The future of AI-enabled healthcare is filled with promise and potential. Through thoughtful collaboration, ethical considerations, and innovative applications of Generative AI, we can unlock new frontiers in personalized medicine, disease prevention, and healthcare delivery. By embracing the opportunities and addressing the challenges, we can realize a future where AI-driven technologies empower patients, support healthcare providers, and transform the way we deliver and experience healthcare.