Can AI Replace Teachers in Schools?
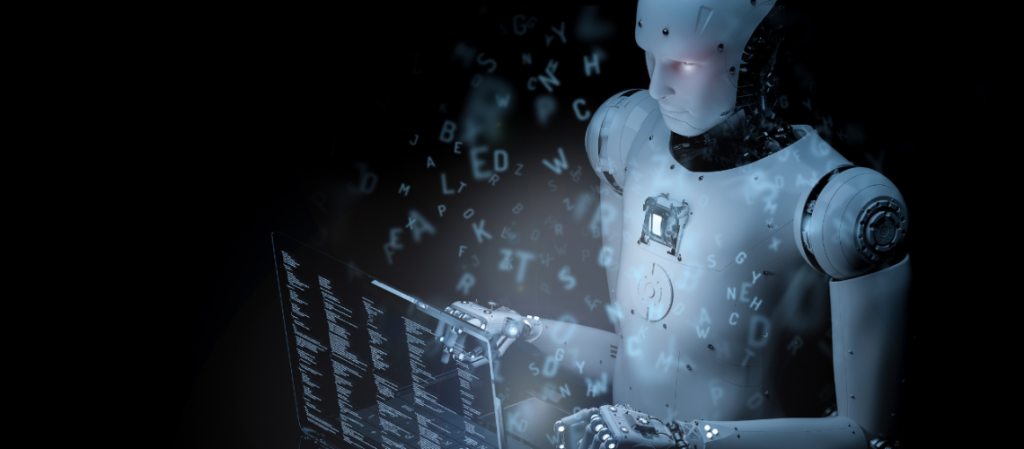
While AI has made significant advancements, can AI replace teachers in school?Teachers serve as facilitators of knowledge, guiding students through the learning process and imparting subject-specific expertise. They possess the human qualities necessary for effective education, such as empathy, understanding, and the ability to connect with students on an individual level. Teachers foster a supportive and nurturing environment that promotes not only academic growth but also personal and social development. They are essential in helping kids develop their values, critical thinking abilities, and creativity. Moreover, teachers provide mentorship, guidance, and emotional support, helping students navigate challenges and develop resilience. The teacher-student relationship is a fundamental aspect of education, as teachers serve as role models and mentors, helping students develop a love for learning and a thirst for knowledge. While AI can support and enhance the educational process, it cannot replicate the human connection and the unique qualities that teachers bring to the classroom. Therefore, the importance of teachers in education cannot be underestimated, as they shape the minds and lives of students in profound and meaningful ways. Advancements in AI technology in Education AI has made significant advancements in recent years, empowering machines with impressive capabilities. AI systems can learn from data and get better over time with the use of machine learning algorithms, which eliminate the need for explicit programming. AI can comprehend and produce human language thanks to natural language processing, which improves interaction and communication. AI systems can recognise objects and comprehend images thanks to computer vision, which enables the interpretation and analysis of visual data. Robotics allows machines to carry out activities in the physical world by fusing AI with mechanical systems. These characteristics have created a wide range of opportunities for the application of AI across many fields, including education. Such as virtual reality (VR) and augmented reality (AR) in education where students can explore virtual environments, engage in simulations, and visualize abstract concepts, making learning more interactive and engaging. Also, data analytics for student performance where AI algorithms can analyze vast amounts of student data, identifying patterns and trends to gain insights into individual and group performance. Potential Benefits of AI in the Classroom Personalized Learning Experiences By customizing instruction and content to each student’s particular requirements and learning preferences, artificial intelligence (AI) offers personalized learning experiences. This customization enhances engagement, understanding, and knowledge retention, as students receive tailored support and challenges based on their abilities and preferences. Efficient Administrative Tasks When administrative activities like scheduling, grading, and record-keeping are automated by AI, teachers have more time to devote to lesson planning, student interactions, and individualized support. This automation streamlines administrative processes, leading to increased efficiency in school operations. Accessible Education for All AI has the potential to bridge gaps in education by providing access to quality resources and instruction for students in underserved areas or with special needs. AI-powered tools can deliver content in different formats, accommodate diverse learning styles, and offer support for students with disabilities, ensuring inclusive education. Enhanced Student Engagement Gamification, virtual reality, and other AI-based technologies can increase student motivation and engagement. By making learning more interactive, immersive, and enjoyable, AI helps students stay actively involved in their education, leading to improved learning outcomes. Limitations of AI in Education Lack of Emotional Intelligence AI systems lack the emotional intelligence that human teachers possess. They cannot fully understand and respond to students’ emotions, which can impact the emotional support and connection that students may need during their learning journey. Emotional intelligence is crucial in creating a supportive and nurturing environment that fosters student well-being and social-emotional development. Limited Ability to Adapt to Individual Needs Although AI can provide personalized learning experiences to some extent, its ability to truly understand and adapt to the unique needs of each student is limited. AI algorithms primarily rely on data-driven patterns and may struggle to capture the complexity and nuances of individual student characteristics, strengths, and challenges. Human teachers, on the other hand, can employ their expertise, intuition, and experience to tailor instruction and support to each student effectively. Ethical Concerns and Privacy Issues The use of AI in education raises questions about privacy and ethics. Massive volumes of data, including student personal information, are gathered and processed by AI systems. Ensuring the security and responsible use of this data becomes crucial to safeguard student privacy and protect against potential misuse or unauthorized access. Ethical considerations also arise regarding bias in AI algorithms and the potential for reinforcing existing inequalities in education. Impact on Social Interactions and Relationships AI’s increasing presence in the classroom may impact social interactions and relationships among students. Human interaction, collaboration, and communication skills are vital aspects of education that contribute to the development of social competence and interpersonal skills. Over Reliance on AI systems may inadvertently reduce opportunities for face-to-face interaction and hinder the development of these important skills. The Role of Teachers in the AI Era Unique Human Qualities and Skills In the AI era, the role of teachers becomes even more crucial. Teachers possess unique human qualities, such as empathy, intuition, and understanding, that are essential for effective education. These qualities allow teachers to connect with students on a deeper level, understand their individual needs, and provide the necessary support and guidance throughout their learning journey. Fostering Critical Thinking and Creativity Teachers play a vital role in fostering critical thinking skills and nurturing students’ creativity. They teach kids to think critically, evaluate data, work through issues, and use higher-order thinking skills. Teachers provide the guidance and space for students to explore their creativity, develop innovative ideas, and express themselves through various mediums. Emotional Support and Mentorship Human teachers offer emotional support and mentorship to students, which AI systems cannot replicate. Teachers provide a safe and nurturing environment where students can seek guidance, share their concerns, and develop social-emotional skills. They serve as mentors, helping students set goals, navigate challenges, and develop resilience. Collaboration with AI for Effective Education Rather than replacing teachers, AI can complement and
Can AI be Used by Teachers in Education?
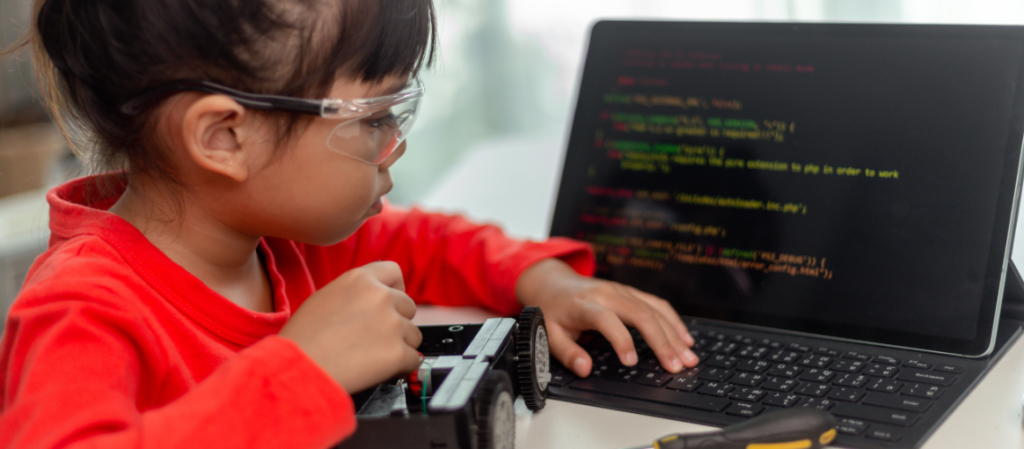
Can AI be used by teachers in education?AI has the ability to completely change how teachers and students interact with the learning process as a result of the expanding role of technology in education. AI offers a variety of applications that can improve educational outcomes, from personalized learning experiences to intelligent tutoring systems. As technology continues to advance, exploring the integration of AI in education becomes increasingly crucial for fostering effective teaching and learning practices. The Potential of AI in Education Personalised Learning 1. Adaptive Assessments and Feedback AI has the capacity to revolutionize personalized learning by adapting educational experiences to individual students’ needs. Artificial intelligence (AI)-powered adaptive assessments and feedback systems can evaluate students’ knowledge and skills in real-time, enabling customized feedback and targeted interventions. 2. Tailoring Content to Individual Needs Through intelligent algorithms, AI can analyse students’ performance data, identify their strengths and weaknesses, and provide personalized recommendations to enhance their learning journey. Additionally, AI can customize content delivery, providing students with resources and materials that align with their specific learning styles and preferences. Intelligent Tutoring Systems 1. Virtual Tutors and Interactive Learning Experiences Intelligent pedagogical systems make use of AI to create virtual tutors that provide interactive and adaptive learning experiences. These systems can engage students through personalized instruction, interactive simulations, and real-time monitoring. 2. Real-time Monitoring and Guidance AI-powered virtual tutors can analyze students’ progress, offer guidance, and adjust the learning pace and content to ensure optimal comprehension. With the ability to adapt to individual needs, these systems enhance student engagement and promote deeper understanding of complex concepts. Automated Grading and Feedback 1. Efficient Evaluation of Assignments and Exams AI streamlines the grading and feedback process, saving teachers valuable time. Automated grading systems can evaluate assignments and exams efficiently, ensuring consistent and objective assessment. 2. Timely Feedback for Student Improvement AI-powered feedback systems can provide students with timely and detailed assessments, highlighting areas for improvement and offering suggestions for further learning. Benefits of AI for Teachers Time-saving Data-driven Insights Enhanced Instructional Strategies Addressing Concerns and Challenges Ethical Considerations Balancing AI with Human Interaction Successful AI Integration in Education Professional Development for Teachers Collaboration Between EdTech Companies and Educators Final Thoughts It is imperative to emphasize the crucial role of instructors in the integration of AI in education. While AI offers numerous benefits, it is teachers who bring their expertise, experience, and human touch to the learning environment. By providing professional development opportunities and fostering collaboration, teachers can harness the benefits of AI effectively. Collaboration between EdTech companies and educators further ensures that AI tools and platforms are co-created, incorporating teacher input and feedback. By working hand in hand, teachers and AI systems can create a synergistic learning environment that optimizes student outcomes and prepares them for the challenges of the future.
Math for AI: Building Intelligent Algorithms with Numbers and Patterns
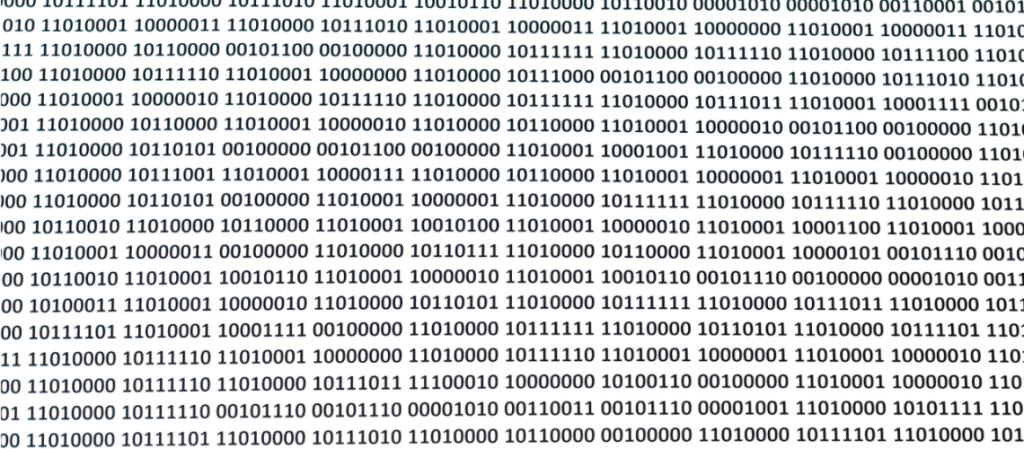
In the modern world, math for AI has emerged as a disruptive force that is altering industries and fostering innovation. In the background, mathematics is essential to the creation and operation of AI systems. Math provides the foundation for AI to analyze data, make informed decisions, and create intelligent algorithms. In this module, we delve into the essential mathematical concepts required for AI, enabling students to grasp the fundamental principles that drive these intelligent systems. Foundation: Laying the Numerical Groundwork At the foundation level, students are introduced to key mathematical concepts that form the basis of AI. They develop number sense, learning to work with numbers confidently and understand their relationships. Multiplication and division become tools for problem-solving, while basic geometry helps students visualize and manipulate shapes. Additionally, the module covers fractions, measurements, and pattern recognition, providing a solid mathematical foundation for AI exploration. Level 1: Deepening Mathematical Understanding At Level 1, students delve into more complex mathematical operations, enhancing their ability to work with larger numbers. They gain a deeper understanding of fractions and are introduced to decimals, broadening their mathematical toolkit. Basic concepts of percentages enable them to analyze data in real-world scenarios. Algebra concepts come into play, laying the groundwork for problem-solving and logical thinking. Students also explore basic data handling and begin to grasp the concept of area and perimeter of simple shapes. Level 2: Exploring Mathematical Relationships Building upon the knowledge gained in Level 1, Level 2 delves into pre-algebra, bridging the gap between arithmetic and algebra. Students are introduced to probability, understanding the likelihood of events and their impact on decision-making. More complex fractions and decimals deepen their numerical fluency. Introduction to ratios and proportions enables them to compare quantities and understand their relationships. They also explore integer operations and dive deeper into basic geometry, including concepts of area, volume, and angles. Level 3: Advancing Mathematical Proficiency Level 3 marks the culmination of mathematical learning for AI. Pre-algebra concepts are reinforced, strengthening students’ problem-solving skills. Geometry takes a more comprehensive turn, exploring advanced topics such as trigonometry. To comprehend data trends and derive valuable insights, probability and statistics become essential. Finally, students are introduced to calculus concepts, providing them with a powerful tool for analyzing change and optimization—a cornerstone of AI development. Conclusion: Unlocking the Power of Math in AI Mathematics serves as the backbone of artificial intelligence, enabling systems to analyze data, learn from patterns, and make informed decisions. The Math for AI module equips students with the necessary mathematical skills at each level, providing a solid foundation for understanding and contributing to the AI field. By mastering concepts like number sense, algebra, geometry, and probability, students gain the ability to build intelligent algorithms and contribute to the ongoing advancements in AI. The next generation is genuinely empowered by maths to realize the possibilities of artificial intelligence and influence a world powered by intelligent technologies.
What is Natural Language Understanding and Natural Language Generation?
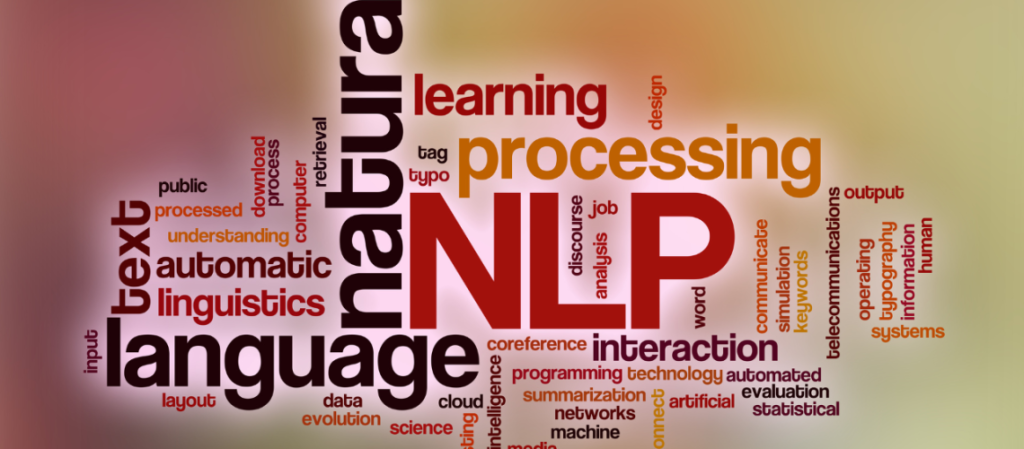
Natural Language Understanding and Natural Language Generation are two vital components of natural language processing that enable machines to interact with human language in a meaningful way. NLU involves the comprehension and interpretation of human language by machines, allowing them to extract meaning, identify entities, and understand user intent. NLG, on the other hand, focuses on producing writing or language that is human-like and is based on structured data or information. Together, NLU and NLG form the backbone of intelligent systems, enabling applications such as virtual assistants, chatbots, automated report writing, and personalized content generation. They play a crucial role in bridging the gap between humans and machines, facilitating effective communication and enhancing user experiences. Natural Language Understanding (NLU) A. Explanation of NLU Natural Language Understanding (NLU) involves the ability of a computer or machine system to comprehend and interpret human language. NLU aims to extract meaning from text or speech by analyzing syntactic and semantic structures, identifying entities and relationships, understanding context, and determining user intent. It enables machines to understand and respond appropriately to human queries or commands. B. Techniques and Approaches in NLU C. Applications of NLU Natural Language Generation (NLG) A. Explanation of NLG The process of creating human-like language or text by a computer or mechanical device is known as natural language generation (NLG). NLG systems take structured data or information as input and transform it into coherent and contextually appropriate sentences, paragraphs, or longer narratives. NLG techniques aim to mimic human language generation, making the generated text indistinguishable from text written by humans. B. Techniques and Approaches in NLG C. Applications of NLG Relationship between NLU and NLG A. NLU as input for NLG Natural Language Generation (NLG) uses Natural Language Understanding (NLU) as an input. NLU helps in interpreting and understanding the input language, extracting key information, identifying entities, and determining user intent. B. NLG as output of NLU NLG can be the output of NLU systems. Once NLU processes and understands the input language, it can generate structured data or information that can be used as input for NLG. This structured data, along with additional context, can be transformed into human-like language or text by NLG systems. C. Examples of NLU and NLG working together NLU and NLG often work together in conversational systems like chatbots or virtual assistants. NLU helps in understanding user queries or commands, extracting the relevant information, and determining the user’s intent. This understanding is then used by NLG to generate appropriate and meaningful responses. For example, when a user asks a virtual assistant, “What is the weather like today?”, NLU identifies the user’s intent as a weather inquiry and extracts the location. NLG then generates a response like, “Today’s weather in [location] is sunny with a high of 25°C.” Challenges and Future Directions A. Challenges in NLU B. Challenges in NLG C. Advances and future directions in NLU and NLG Conclusion The future of NLU and NLG holds promising possibilities. Advancements in language models, contextual understanding, and multimodal integration can lead to more accurate and natural language processing systems. Future developments may focus on overcoming challenges related to ambiguity, bias, and context adaptation. Additionally, ethical considerations and responsible AI practices will play a crucial role in ensuring fairness, transparency, and inclusivity in NLU and NLG systems. Continued research and innovation in these areas will unlock new potentials and applications for NLU and NLG, benefiting various industries and transforming human-machine interactions.
Can We Make a Machine Intelligent?
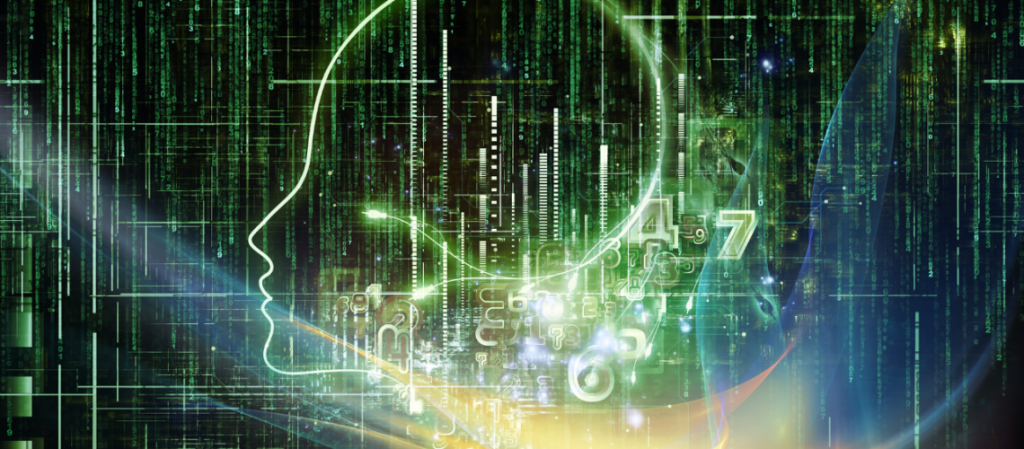
The idea of machine intelligence has captivated the imaginations of academics, researchers, and the general public alike in today’s quickly evolving technological landscape. Can we really create an intelligent machine given the tremendous developments in computer power, algorithms, and data accessibility? We will examine the complexities of artificial intelligence in this blog, as well as its potential, difficulties, and ethical issues. The ability of a computer or other machine to carry out tasks that ordinarily require human intelligence is referred to as machine intelligence. It entails the creation of algorithms and systems that are able to comprehend, reason, learn, and make judgements based on patterns and data. Computer vision, robotics, natural language processing, and machine learning are just a few of the subfields that make up machine intelligence. Types of Machine Intelligence There are many different types of machine intelligence, and each has its own strengths and weaknesses. Let’s explore some common categories of machine intelligence: History of Machine Intelligence Since the beginning of computing, researchers have been trying to create artificial intelligence. The field gained prominence in the 1950s and 1960s with the development of symbolic AI, which aimed to create intelligent machines by using predefined rules and logical operations. However, symbolic AI faced limitations in handling real-world complexities. Subsequently, machine learning emerged, focusing on the development of algorithms that allow machines to learn from data and improve their performance over time. Machine Intelligence Developments A recent surge in machine intelligence has been fueled by improvements in computer power, algorithms, and data accessibility. Let’s look at some of the main developments in artificial intelligence: Challenges in Achieving Machine Intelligence While machine intelligence has made remarkable progress, several challenges persist on the path to achieving truly intelligent systems. Let’s delve into some of the key challenges: Applications of Machine Intelligence Machine intelligence finds application in various domains, transforming industries and enhancing human capabilities. Let’s look at some of the major fields where artificial intelligence is having a big impact: Future Prospects of Machine Intelligence The future of machine intelligence holds immense possibilities. Continued advancements in machine learning, coupled with interdisciplinary research, will lead to breakthroughs in areas such as natural language understanding, robotics, and cognitive computing. To ensure the ethical and inclusive development of intelligent machines, it is crucial to address societal and ethical issues. Conclusion The question of whether we can make a machine intelligent is a complex one. While machines have made remarkable strides in simulating human-like intelligence, they are still far from replicating the full spectrum of human cognitive abilities. However, with ongoing research and advancements, the boundaries of machine intelligence continue to expand. It is crucial to navigate the challenges and ethical considerations associated with this technology to harness its potential for the benefit of humanity.
Explore Machine Learning and How It Works
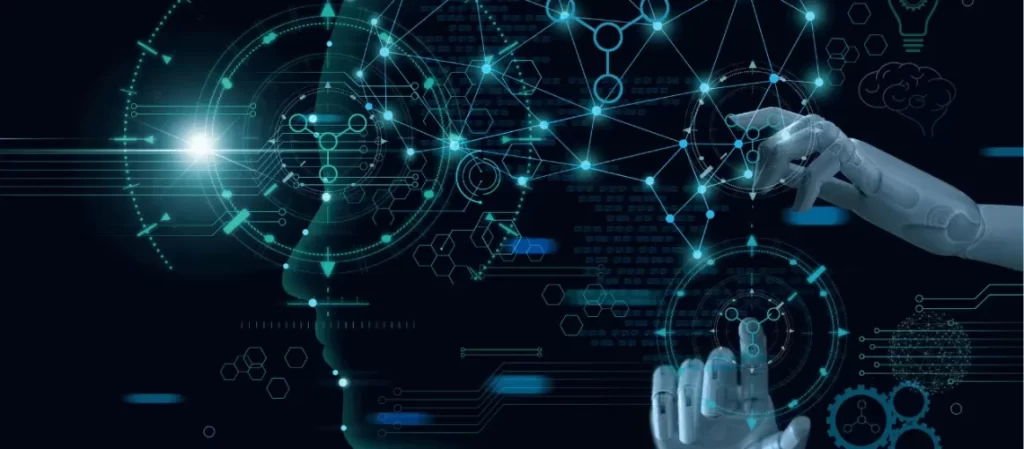
A subfield of artificial intelligence (AI) called “machine learning” focuses on creating models and algorithms that let computers infer conclusions from data without having to be explicitly programmed. In other words, it’s a field that gives machines the ability to gain knowledge from their mistakes and develop over time. Machine learning has gained immense importance due to its ability to analyze vast amounts of data, extract meaningful insights, and automate complex tasks. It has uses across a range of sectors, including: Types of Machine Learning Algorithms A. Supervised Learning The model is trained using labeled data, or input data to which the relevant output labels have been applied, in supervised learning. The objective is for the model to learn the relationship between the input features and the related output labels, enabling it to forecast or categorize brand-new, unexplored data. Tasks that are examples of supervised learning include: In supervised learning, the training process involves presenting the model with labeled examples from the training dataset. The model learns to generalize from these examples and make predictions for unseen data. The input features and their accompanying output labels make up the labeled data. Usually, the process involves splitting the dataset into training and validation sets, with the training set being used to develop the model and the validation set being used to assess its performance. B. Unsupervised Learning Unsupervised learning is a kind of machine learning in which the model discovers structures and patterns in unlabeled data without having to be told what the output labels should be. The objective is to investigate and comprehend the relationships or inherent design in the data. Unlike supervised learning, no known target variable guides the learning process. Instead, the algorithm discovers patterns or groupings on its own. Unsupervised learning tasks examples include: C. Reinforcement Learning An agent is taught how to interact with its surroundings in order to maximize cumulative reward using machine learning techniques like reinforcement learning. The agent gains knowledge by making mistakes and then receiving feedback in the form of incentives or punishments based on its behavior. Reinforcement learning is often used in scenarios where explicit training data is unavailable or impractical. Examples of reinforcement learning applications include: Machine Learning Workflow A. Machine Learning Workflow A key element of machine learning is workflow, which includes a number of phases from data preprocessing to model training and evaluation. Let’s delve into the first stage of the workflow: data preprocessing. 1. Data cleaning and handling missing values Data preprocessing entails preparing and cleaning the raw data to guarantee its uniformity and quality. The treatment of missing numbers, handling of outliers, and correction of any errors or inconsistencies in the data are all included in this stage. Imputation techniques like mean or median imputation, as well as more complex techniques like regression imputation or multiple imputations, are frequently used to handle missing information. 2. Feature scaling and normalization Feature scaling is essential to ensure that all features or variables in the dataset are on a similar scale. This step helps prevent certain features from dominating the learning process due to their larger magnitude. Scaling techniques such as standardization (mean centering and scaling to unit variance) or normalization (scaling to a specific range, e.g., [0, 1]) are commonly applied to achieve this. 3. Feature engineering and selection Feature engineering involves creating new features or transforming existing ones to enhance the model’s predictive power. This step may include mathematical transformations, combining existing features, or extracting relevant information from text or images. The goal is to find important connections or patterns that might not be obvious from the initial raw data. B. Model training and evaluation 1. Creating training and test sets from data Once the data preprocessing stage is complete, the dataset is typically divided into two separate sets: a training set and a testing set. A training set and a testing set are used to train and evaluate a machine learning model, respectively. The splitting of data helps assess how well the model generalizes to unseen data. 2. Training models using algorithms and labeled data A machine-learning model is now trained using a selected method and training set. The input features and accompanying output labels or target values make up the labeled data, which the system learns from. In an effort to reduce the discrepancy between projected and actual outputs, the model modifies its internal parameters or weights based on the training data. This process involves iterations or epochs until the model converges to an optimal state. 3. Evaluating model performance using metrics After training, the model needs to be put to the test to see how well it performs. Evaluation is typically done using metrics that measure various aspects of the model’s predictive capability. Depending on the specific work, common evaluation measures can include: Potential Impact and Future Possibilities Healthcare, banking, transportation, and entertainment are just a few of the industries where machine learning has already had a substantial impact. With ongoing advancements in hardware capabilities and the availability of vast amounts of data, the potential impact of machine learning is expected to grow exponentially. Future possibilities include improved personalized medicine, autonomous vehicles, enhanced natural language processing, and intelligent virtual assistants. Some of the most urgent problems in the world, such as resource optimization, illness prediction, and climate change, can be helped by machine learning. In this exploration of machine learning, we covered the fundamental aspects and workflow involved in this field. We discussed supervised learning, where models are trained using labeled data for prediction and classification tasks. Unsupervised learning, on the other hand, allows models to discover patterns and structures in unlabeled data through techniques like clustering and dimensionality reduction. Last but not least, reinforcement learning enables agents to learn and decide in dynamic environments based on rewards and punishments. Continuous learning is of paramount importance in the field of machine learning. Given the rapid advancements and evolving nature of technology and data, it is crucial for practitioners to stay updated with the
Deep Learning: How It Transforms Our Lives and Boosts AI Advancements
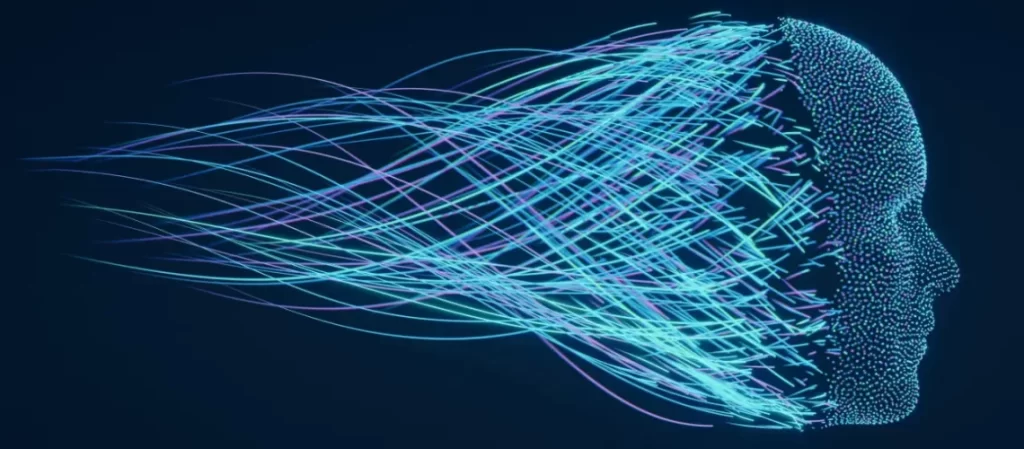
Deep learning, in its simplest terms, is a type of computer technology that tries to make computers think and learn like the human brain. It involves using computer programs called artificial neural networks to process information and make decisions in a way that resembles how our brains work. These networks have many layers of interconnected parts, allowing them to understand and learn from complex data, such as images, text, and sound, without needing direct instructions from humans. Deep learning has been used to solve challenging problems like recognizing objects in photos, understanding spoken language, and even playing complex games, making it a powerful tool in the field of artificial intelligence. Machine Learning Machine learning is a subfield of computer technology a.k.a artificial intelligence (AI) that allows computers to learn and make predictions or decisions without being explicitly programmed to do so. Here’s a basic breakdown for Machine Learning: What does the Term ‘Deep Learning’ Mean? Deep learning is a division of machine learning that involves training an Artificial Neural Network (ANN), that performs various machine learning tasks. In the simplest context, we are teaching a computer to mimic the human brain’s way of learning and solving problems. How does Deep Learning function? Here’s a basic explanation of how deep learning works: In essence, deep learning is about building complex mathematical models (neural networks) that can learn and represent patterns in data. It’s particularly powerful for tasks involving large amounts of data and complex relationships, such as image and Speech Recognition, Natural Language Processing (NPL), and Autonomous Driving. Why is it Called Deep Learning? Deep learning is called “deep” because it employs the use of artificial neural networks with multiple hidden layers between the input and output layers. The term “deep” refers to the depth of these networks, meaning they have many layers, which distinguishes them from shallow networks with fewer hidden layers. The depth of the network is a key characteristic of deep learning and sets it apart from traditional machine learning methods that often use shallower models. The idea behind deep learning is to create more complex and hierarchical representations of data by allowing the network to learn increasingly abstract features as it processes information through these deep layers. The use of deep neural networks has led to significant advancements in various fields, including computer vision, natural language processing, speech recognition, and more, as they are highly effective at capturing intricate patterns and representations in data. Hence, the name “deep learning” reflects the fundamental architectural feature of these neural networks, which is their depth. How many layers does a neural network need to be considered “deep learning”? In the context of deep learning, “depth” simply means the number of layers in a neural network. When we say a network is “deep,” it means it has more than just a couple of layers; it typically has several hidden layers between the input and output layers. However, there’s no specific number of layers that makes a network “deep.” Instead, it’s about having enough layers to learn complex patterns and representations from data. So, the more layers, the better a network can capture intricate details and make sense of complex information, like recognizing objects in images or understanding language. But, it’s also important to balance depth with computational resources and data availability. Advantages of Deep Learning: Unlocking the Power of Neural Networks Deep learning offers several advantages, which have contributed to its widespread adoption and success in various fields. Some of the key advantages include: Applications of Deep Learning in Everyday Life Deep learning is used in our daily lives in various ways. One relatable application is in the recommendation systems used by streaming platforms like Netflix or YouTube. When you see personalized movie or video recommendations, deep learning is at work. These systems analyze your past viewing habits, preferences, and even what other users with similar tastes have watched. Then, they use deep learning algorithms to suggest content you’re likely to enjoy, making your streaming experience more engaging and convenient. Deep Learning vs. Machine Learning: What Sets Them Apart? Deep learning and machine learning are related fields, but the main difference is in the type of algorithms they use it. Deep Learning: A Key Component in the AI and Machine Learning Framework Deep learning is a specialized subset of machine learning, and machine learning is a part of the broader field of artificial intelligence (AI). While AI encompasses various techniques to create intelligent systems, machine learning focuses on algorithms that enable computers to learn from data. Deep learning, within machine learning, uses deep neural networks to automatically learn complex patterns from data, making it particularly effective in AI applications like image recognition and natural language understanding. In essence, deep learning is a powerful tool within the realm of AI and machine learning. Drawbacks Despite these advantages, it’s important to note that deep learning also comes with challenges, such as the need for large amounts of labeled data, computational resources, and potential interpretability issues for complex models. Nevertheless, its benefits have made it a transformative technology in fields like computer vision, natural language processing, and healthcare, among others.
50 ChatGPT Prompts for Math Teachers
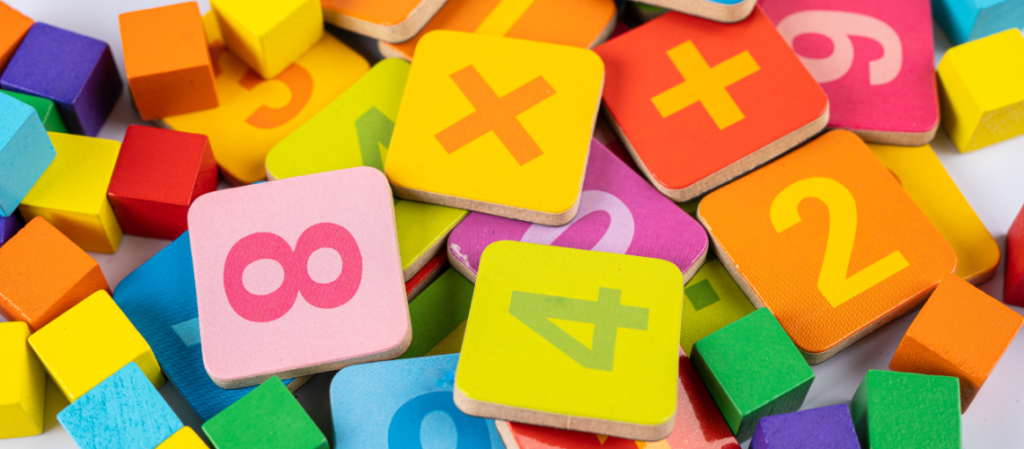
ChatGPT prompts for math teachers can be used to stimulate discussions, provide feedback, and engage students in critical thinking. The prompts cover a range of math topics, from basic arithmetic to calculus and statistics, and can be used to differentiate instruction and provide personalized feedback. From calculating our finances to addressing challenging technical challenges, mathematics is a subject that is fundamental to our lives. Math teachers play a critical role in shaping students’ understanding and love for mathematics. However, teaching math can be challenging, especially when students struggle with the subject or find it uninteresting. To address this challenge, teachers need innovative teaching strategies that can engage and motivate their students to learn math effectively. We’ll give maths teachers 50 ChatGPT prompts in this blog post. These prompts are designed to inspire teachers to explore different teaching strategies and help students learn math in fun and exciting ways. We will cover a range of topics, from basic math to advanced calculus, and provide practical tips and strategies for teaching each concept effectively. We hope that these prompts will help math teachers enhance their teaching skills, provide more personalized instruction, and help students develop a deeper understanding of mathematics. 50 ChatGPT Prompts for Math Teachers Basic Math 1. How can young toddlers be taught addition and subtraction? 2. What inventive methods can you use to teach division and multiplication? 3. How can you make fractions more understandable for students? 4. What are some strategies for teaching decimals? 5. How can you make math fun for kids who struggle with it? Algebra 6. How can you help students understand the concept of variables? 7. What are some successful approaches to resolving linear equations? 8. How can you teach students to graph linear equations? 9. What are some tips for teaching quadratic equations? 10. How do you teach algebra using examples from the real world? Geometry 11. How can you introduce lines and angles to your students? 12. How can geometric changes be explained to students? 13. What approaches can be used to teach pupils about polygons? 14. How can you use manipulatives to teach geometry? 15. How can you make geometry more interesting for students? Trigonometry 16. What are some effective ways to teach trigonometry? 17. How can you aid pupils in comprehending how sine, cosine, and tangent relate to one another? 18. Which methods can be used to solve trigonometric equations? 19. How can you teach students to use trigonometry in real-world situations? 20. How can you make trigonometry more engaging for students? Calculus 21. How can you introduce calculus to high school students? 22. What are some effective strategies for teaching limits? 23. How can you teach students to differentiate and integrate functions? 24. What are some tips for solving optimisation problems? 25. How may calculus be taught using examples from the real world? Statistics 26. How can data collection and analysis be taught to students? 27. What are some successful methods for imparting probability? 28. How can you help students understand the concept of statistical inference? 29. What are some tips for teaching regression analysis? 30. How can statistics be taught using examples from the actual world? Miscellaneous 31. How can instruction be tailored for pupils with various learning preferences? 32. What are some effective strategies for teaching problem-solving skills? 33. How can you assist pupils who are afraid of maths? 34. What are some tips for teaching students to check their work? 35. How can technology be used to improve maths instruction? 36. How can you teach pupils to use mathematical ideas in real-world contexts? 37. How can you help students understand the importance of math in their future careers? 38. What are some successful teaching methods for mathematical modelling? 39. How can you teach students to use logic and reasoning in math? 40. How can you incorporate real-life examples in math lessons? 41. How can you teach students to estimate solutions to math problems? 42. How can you teach students to use mental math strategies? 43. What are some effective strategies for teaching math vocabulary? 44. How can you help students develop their mathematical reasoning skills? 45. How can you teach students to use diagrams and visual aids in math? 46. How can you help struggling students catch up with the class? 47. How can you challenge advanced students in math? 48. What are some tips for grading math assignments and tests? 49. How can you involve parents in math education? 50. What are some effective strategies for providing feedback to students in math? These 50 ChatGPT prompts for math teachers provide a wide variety of ideas and strategies for engaging students in mathematics. From incorporating real-life applications to using technology to fostering a growth mindset, these prompts offer creative and effective ways to make math more accessible and enjoyable for all students. By implementing these ideas, math teachers can help students develop a deeper understanding and appreciation of math, and inspire them to pursue careers in STEM fields. With continued innovation and experimentation, math education can become even more effective, and students can develop the skills they need to succeed in an ever-changing world.
Navigating How Schools and AI Can Coexist for a Brighter Future
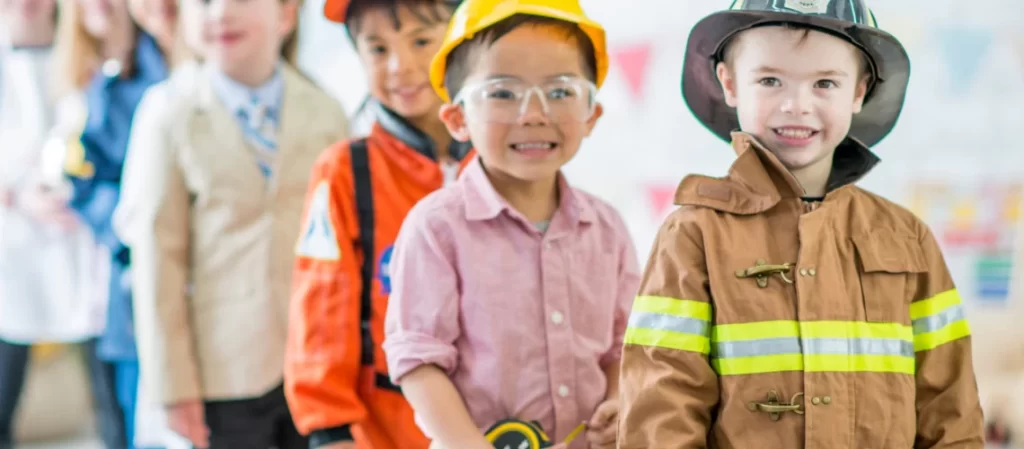
Education has always been a dynamic field, continually adapting to changing societal needs and technological advancements. Over the centuries, we’ve witnessed the transition from traditional classroom-based learning to the digital age of education. However, the most transformative phase is upon us now, driven by the rapid integration of Artificial Intelligence (AI) into the education sector. In the past few years, we have witnessed the transformative power of AI, reshaping nearly every facet of our lives. Particularly, the education sector has undergone significant upgrades. The AI revolution in education represents a seismic shift in how we teach and learn. It encompasses a wide array of applications, from intelligent tutoring systems that personalize learning experiences to data analytics that offer unprecedented insights into student performance. As anticipated, the adoption of Artificial Intelligence (AI) in U.S. education has seen a substantial increase of 45%. This trend is expected to continue, with the AI market in the U.S. education sector projected to grow by an estimated USD 1,100.07 million between 2022 and 2027, according to the Artificial Intelligence Market in the US Education Sector. This growth is marked by a significant focus on Intelligent Tutoring Systems (ITS), which can be likened to having a smart computer program or software that assists students in their learning journey. ITS can assess students’ knowledge levels and provide personalized lessons and feedback, akin to having a dedicated tutor on one’s computer. Furthermore, the integration of 24×7 available chatbots and AI virtual Assistants within universities is a key driver of this market expansion. Utilizing Artificial Intelligence (AI) in education is not merely a trend; it’s a fundamental transformation that holds the promise of creating more efficient, effective, and inclusive learning environments. As we delve deeper into this blog, we’ll explore the myriad ways AI is impacting education, from enhancing personalized learning experiences to addressing longstanding challenges in the education system. Let’s embark on a journey to understand how schools and AI can coexist, ushering in a brighter future for learners of all ages. Top 13 Benefits of AI in Education The benefits of AI in education are numerous and can significantly enhance the learning process. Here are some key advantages: 1. Personalized Learning AI can tailor learning experiences as per individual students requirements by analyzing their strengths and weaknesses. It can adapt content, pace, and difficulty levels to match each student’s unique needs, promoting more effective learning. For example, Khan Academy, an online learning platform, uses AI algorithms to adapt lessons in real-time based on a student’s progress. It tailors the content and difficulty level, ensuring that learners receive personalized instruction. 2. Efficiency AI-powered systems can automate administrative tasks, such as grading assignments and managing schedules. This allows educators to focus more on teaching and providing personalized support to students. One of the best examples here is The Georgia Institute of Technology, uses an AI chatbot named Jill Watson to handle routine administrative inquiries from students. It frees up staff time for more complex tasks and student interactions. 3. Immediate Feedback AI can provide instant feedback on assignments and assessments, helping students identify and correct mistakes in real-time. This timely feedback enhances understanding and retention of concepts. For instance, a language learning app, called Duolingo, provides instant feedback on pronunciation and exercises. If a user makes a mistake, the app corrects them immediately, helping users improve their language skills. 4. Accessibility AI can make education more accessible to individuals with disabilities by providing features like text-to-speech, speech recognition, and closed captioning, ensuring that all students can participate fully in the learning process. One such AI application is Microsoft’s Seeing AI app, which uses AI to provide audio descriptions of the environment to blind or visually impaired users. This empowers them to participate fully in educational settings and everyday activities without compromising. 5. Data-Driven Insights AI can analyze large volumes of educational data to identify trends and patterns. Educators can use these insights to make informed decisions about curriculum design, teaching strategies, and student interventions which facilitates the entire learning process and takes it to the next level. One such application is employed by The Summit Learning Program to analyze student data and provide insights to teachers. Educators can then adjust their teaching strategies to address specific student needs effectively and efficiently. 6. Cost Savings Automating certain tasks through AI can reduce the overall cost of education. This includes savings on administrative expenses and the potential for lower textbook costs through AI-generated educational materials. For instance, The University of Arizona reduced textbook costs by 66% by using an AI-driven platform called Acrobatiq to create personalized course materials. This saved students money and improved access to course materials. 7. Non-stop Availability AI-powered educational tools are available 24/7, allowing students to learn at their own pace and on their own schedule, which can be especially beneficial for adult learners and those with busy lifestyles. Coursera, an online education platform, offers courses that learners can access anytime. This flexibility allows working adults and individuals with busy schedules to pursue education at their convenience. 8. Customized Content Creation AI can generate customized educational content, such as quizzes, exercises, and study materials, based on individual learning needs and preferences. One of the AI-powered tools named ScribeSense can generate customized math worksheets for students. Teachers can create materials curated according to each student’s proficiency level and learning style. 9. Language Learning AI-powered language learning apps can provide immersive language practice through speech recognition, language translation, and conversation simulations, accelerating language acquisition. For instance, Babbel, a language learning app, uses AI to provide interactive language lessons. Users can practice speaking with the app, which uses speech recognition to provide feedback and improve pronunciation. 10. AI Assisting Teachers Artificial Intelligence can assist teachers by providing them with resources, suggesting lesson plans, and offering insights into student progress, thereby making their job more efficient and effective. For example, Carnegie Learning’s MATHia software assists math teachers by providing real-time insights into student progress. It suggests lesson plans and strategies based on individual student needs. 11. Utilizing Learning Management Systems (LMS) powered by AI Learning Management Systems (LMS) are effective tools that allow students to practice problems independently after learning sessions, assessing their comprehension in the
2024: The Year AI, Multimodal Learning, and the Industrial Metaverse Redefine Reality
In 2024, the AI and Machine Learning landscape is poised for a groundbreaking transformation. This revolution is driven by rapid advancements in AI software, including ChatGPT, Multimodal Learning, and the emergence of the Industrial Metaverse. These innovations are set to redefine industries and reshape the way we interact with technology, ushering in a new era of unprecedented possibilities. What is Artificial Intelligence? As the name implies, it is the creation of artificial intelligence by humans to mimic human intelligence using machines and softwares. Artificial Intelligence refers to the field of computer science dedicated to creating machines and software systems that replicate and simulate human-like intelligence and cognitive abilities. These AI systems are designed to perform tasks, solve problems, and make decisions, often with the goal of achieving human-level or superhuman-level performance in various domains. What is Machine Learning? Machine learning is a type of computer technology that allows machines to learn from data and improve their performance on specific tasks without being directly programmed. In essence, it’s a way for computers to get better at tasks by recognizing patterns in data, much like how humans learn from experience. What is Multimodal Learning in a simple context? Multimodal learning, in simple terms, is the process of teaching and learning using multiple modes or types of information simultaneously. This means using various sources of data, such as text, images, videos, and audio, to gain a deeper and more comprehensive understanding of a topic or concept. It’s like learning from different angles to get a more complete picture. Which domains are using Multimodal Learning as Technology to advance? Multimodal learning is becoming increasingly important in various applications and technologies. Here are two case studies: Case Study 1: Healthcare In the field of healthcare, Multimodal Learning is revolutionizing medical imaging. By combining data from different medical imaging techniques such as X-rays, MRIs, and CT scans, doctors can improve diagnostic accuracy significantly. This fusion of data allows for a more precise and comprehensive view of a patient’s condition, leading to better treatment decisions and outcomes. Case Study 2: Education Multimodal learning is also making waves in education. Interactive e-learning platforms now integrate text, videos, and interactive simulations to offer diverse learning materials. This approach caters to different learning styles and enhances comprehension, making education more engaging and effective. These are just a few examples, but the concept of multimodal learning is expanding across many domains as technology continues to advance while significantly speeding human lives and transforming industries. What is the emergence of the Industrial Metaverse? The “Industrial Metaverse” is a term used to describe the convergence of digital technologies, virtual environments, and data analytics within industrial and manufacturing sectors. It represents the application of metaverse concepts (originally associated with virtual worlds and augmented reality) to enhance and transform various aspects of industry and manufacturing. Here’s a simplified explanation for the emergence of the Industrial Metaverse refers to the growing adoption of virtual and digital technologies in industrial settings, In essence, the Industrial Metaverse represents a shift toward a more digitized and interconnected industrial landscape, where virtual and digital technologies are used to enhance productivity, efficiency, and innovation across multiple industrial sectors such as energy, manufacturing, transportation, and more. It’s a vision of a highly interconnected and data-driven industrial future. Related Terminology Augmented Reality: Augmented reality (AR) is like a magic window into the real world. It uses technology to add computer-generated things, like pictures, videos, or information, to what you see around you. Imagine looking at your smartphone’s camera, and on the screen, you see virtual objects that appear to be part of the real world. That’s AR – mixing the real world with digital stuff to make your experience more interesting or useful. Here’s a real-life example of augmented reality, imagine you’re walking down the street with your smartphone. You open a navigation app, and instead of just seeing a map, your phone’s camera shows you the actual street view. However, it also adds arrows and signs directly onto the screen, overlaid on the real-world scene, guiding you to your destination as you move. These digital arrows and signs are not really there in the physical world; they are part of the augmented reality provided by the app. This helps you find your way more easily in the real world with the assistance of digital information. Natural Language Processing (NLP): This is a subfield of AI a.k.a Artificial Intelligence that focuses on the interaction between computers and human language by breaking down the language barrier into a format that can be comprehend and used by both humans and computers. NLP precisely enables machines to understand, interpret, and generate human language. A real-time example of Natural Language Processing (NLP) in action is the use of chatbots in customer service. Many companies employ AI-powered chatbots on their websites or messaging platforms to interact with customers. When a customer types a question or issue in natural language, the chatbot uses NLP algorithms to analyze and understand the text. It then generates a relevant response or takes appropriate actions based on the customer’s query. This interaction happens in real-time, allowing customers to get assistance or information immediately, even outside of regular business hours. For instance, if you visit a retail website and ask a chatbot, “Can you help me find a pair of black running shoes in size 9?”, the NLP-driven chatbot will process your request, understand the keywords and intent (“find black running shoes in size 9”), and provide you with product recommendations or direct you to the relevant product pages. Deep Learning: This is also one of the subfields of AI. It is a type of computer technology that tries to make computers think and learn like the human brain. It involves using computer programs called artificial neural networks to process information and make decisions in a way that resembles how our brains work. These networks have many layers of interconnected parts, allowing them to understand and learn from complex data, such as images, text, and sound, without needing direct instructions