The idea of machine intelligence has captivated the imaginations of academics, researchers, and the general public alike in today’s quickly evolving technological landscape. Can we really create an intelligent machine given the tremendous developments in computer power, algorithms, and data accessibility? We will examine the complexities of artificial intelligence in this blog, as well as its potential, difficulties, and ethical issues.
The ability of a computer or other machine to carry out tasks that ordinarily require human intelligence is referred to as machine intelligence. It entails the creation of algorithms and systems that are able to comprehend, reason, learn, and make judgements based on patterns and data. Computer vision, robotics, natural language processing, and machine learning are just a few of the subfields that make up machine intelligence.
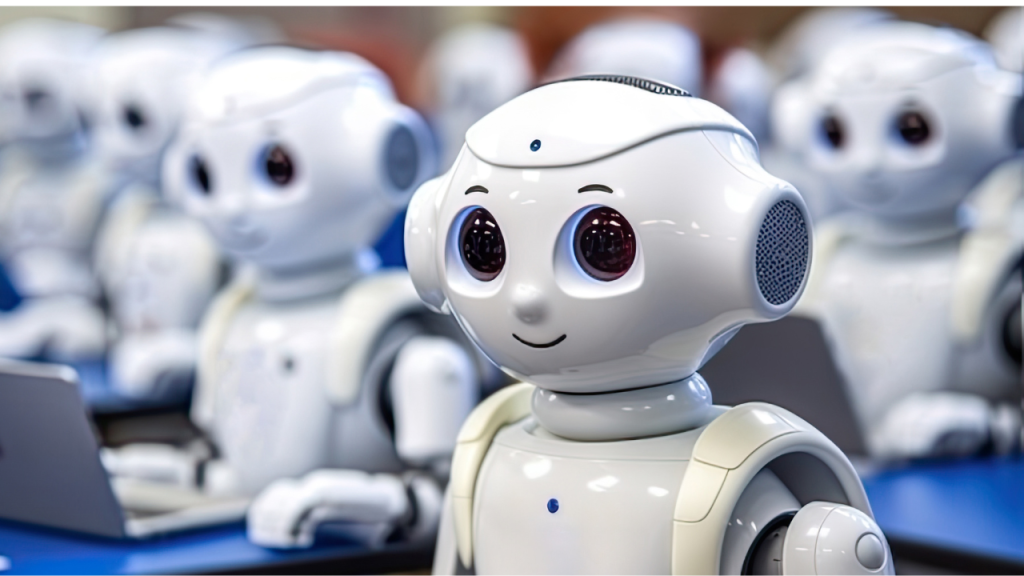
Types of Machine Intelligence
There are many different types of machine intelligence, and each has its own strengths and weaknesses. Let’s explore some common categories of machine intelligence:
- Narrow AI (Weak AI): Narrow AI refers to systems built to carry out particular jobs expertly. These AI systems excel in specialized areas such as voice recognition, image classification, or recommendation engines.
- General AI (Strong AI): General AI, on the other hand, strives to achieve an intelligence level that is comparable to or greater than that of humans across a variety of fields. Such AI systems would not only excel in specific tasks but would also have the ability to understand, learn, and apply knowledge across a range of tasks.
- Superintelligent AI: Superintelligent AI represents the pinnacle of machine intelligence, surpassing human cognitive abilities in virtually every domain. Excellent problem-solving abilities, creativity, and even self-awareness would be present in this level of AI. However, the development of such advanced AI systems remains speculative, and there are considerable debates and concerns regarding their implications and control.
- Machine Learning-based AI: Over time, ML-based AI systems get better at what they do by learning from data. Based on the data they are exposed to, they are able to recognise patterns, forecast the future, and modify their behavior. Supervised learning, unsupervised learning, and reinforcement learning are some common techniques within machine learning that contribute to the development of intelligent systems.
- Natural Language Processing (NLP): NLP aims to give computers the ability to comprehend and decipher human language. It involves techniques such as speech recognition, sentiment analysis, language translation, and question-answering systems. NLP enables more intuitive and natural communication between robots and people.
- Computer Vision: With the use of computer vision, machines can recognise and decipher visual data from pictures or movies. It involves tasks such as object detection, image classification, facial recognition, and scene understanding. Applications like driverless vehicles, surveillance systems, and medical imaging all heavily rely on computer vision.
History of Machine Intelligence
Since the beginning of computing, researchers have been trying to create artificial intelligence. The field gained prominence in the 1950s and 1960s with the development of symbolic AI, which aimed to create intelligent machines by using predefined rules and logical operations. However, symbolic AI faced limitations in handling real-world complexities. Subsequently, machine learning emerged, focusing on the development of algorithms that allow machines to learn from data and improve their performance over time.
Machine Intelligence Developments
A recent surge in machine intelligence has been fueled by improvements in computer power, algorithms, and data accessibility. Let’s look at some of the main developments in artificial intelligence:
- Deep Learning: A branch of machine learning, deep learning has transformed AI. Deep learning has shown remarkable performance in tasks such as image and speech recognition, natural language processing, and generative modeling.
- Big Data and Cloud Computing: The proliferation of big data has provided AI systems with vast amounts of information for training and learning. The advent of cloud computing has made it easier to store, process, and analyze this data, accelerating the development of intelligent systems.
- Reinforcement Learning: Reinforcement learning is a branch of machine learning that focuses on training agents to make sequential decisions in an environment. This has produced innovations in fields including gaming, robotics, and autonomous systems.
- Transfer Learning: Transfer Learning enables artificial intelligence (AI) systems to use knowledge obtained from one activity and apply it to another task that is related to it. Transfer learning has proven beneficial in scenarios with limited data availability, allowing models to generalize better and achieve higher performance.
- Explainable AI: Explainable AI is concerned with creating methods and algorithms that shed light on how AI systems make decisions. This fosters trust, enables better accountability, and helps address ethical concerns related to biases or unintended consequences.
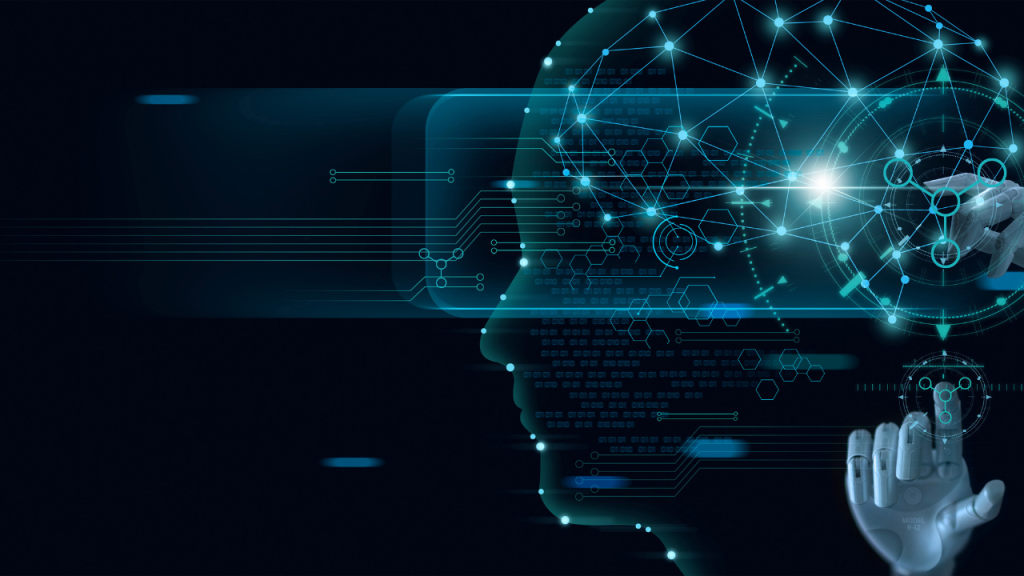
Challenges in Achieving Machine Intelligence
While machine intelligence has made remarkable progress, several challenges persist on the path to achieving truly intelligent systems. Let’s delve into some of the key challenges:
- Data Limitations: Machine intelligence heavily relies on quality data for training and learning. However, obtaining labeled and diverse datasets, particularly for complex tasks, can be challenging. Data scarcity or biases within the data can hinder the performance and generalizability of AI systems.
- Interpretability and Trustworthiness: As AI systems become more sophisticated, their decision-making processes become less transparent. Understanding how AI systems arrive at their conclusions or recommendations is crucial for users to trust and rely on them.
- Ethical Considerations: AI systems must be designed and deployed in ways that prioritize fairness, privacy, and human well-being. Addressing biases in training data, ensuring transparency, and establishing guidelines for responsible AI development are crucial to mitigate ethical challenges and potential societal risks.
- Contextual Understanding and Common Sense: While AI systems have made significant progress in specialized tasks, they often lack the broader contextual understanding and common sense reasoning that humans possess.
- Integration and Adoption: Organizations face challenges in identifying the right use cases, managing the transition, and upskilling their workforce to leverage AI effectively. Widespread adoption of machine intelligence requires addressing technological, organizational, and cultural barriers.
Applications of Machine Intelligence
Machine intelligence finds application in various domains, transforming industries and enhancing human capabilities. Let’s look at some of the major fields where artificial intelligence is having a big impact:
- Healthcare: X-rays, MRIs, and CT scans are just a few examples of the medical imagery that AI systems can analyze. This helps in the early diagnosis of diseases. Chatbots and virtual assistants with AI capabilities improve patient engagement and offer individualized healthcare suggestions.
- Finance: AI systems examine enormous volumes of financial data to spot ominous trends and abnormalities that can stop fraud. Machine learning algorithms predict market trends and optimize trading strategies.
- Transportation: Machine intelligence plays a vital role in the transportation sector, particularly in the development of autonomous vehicles. AI systems process sensor data, interpret traffic patterns, and make real-time decisions, improving road safety and efficiency. Machine learning algorithms optimize route planning and logistics, reducing transportation costs and improving delivery timelines.
- Manufacturing: AI systems analyze sensor data from machinery to identify potential failures and schedule maintenance proactively, minimizing downtime. Systems using machine vision check products for flaws to ensure high quality and cut down on waste. AI algorithms optimize inventory management, demand forecasting, and production planning, enhancing efficiency and reducing costs.
- Customer Service: Based on user preferences and previous information, virtual assistants provide personalized recommendations and bespoke experiences. Sentiment analysis enables businesses to evaluate consumer happiness, spot patterns, and enhance their goods and services in response to client input.
- Entertainment: To deliver individualized suggestions for films, music, and online material, AI systems analyze user interests, watching history, and social media connections. AI-powered content generation assists in tasks such as video editing, automated transcription, and image recognition. Gaming, films, and virtual tours may all be enjoyed in an immersive and participatory way thanks to virtual reality (VR) and augmented reality (AR) technologies.
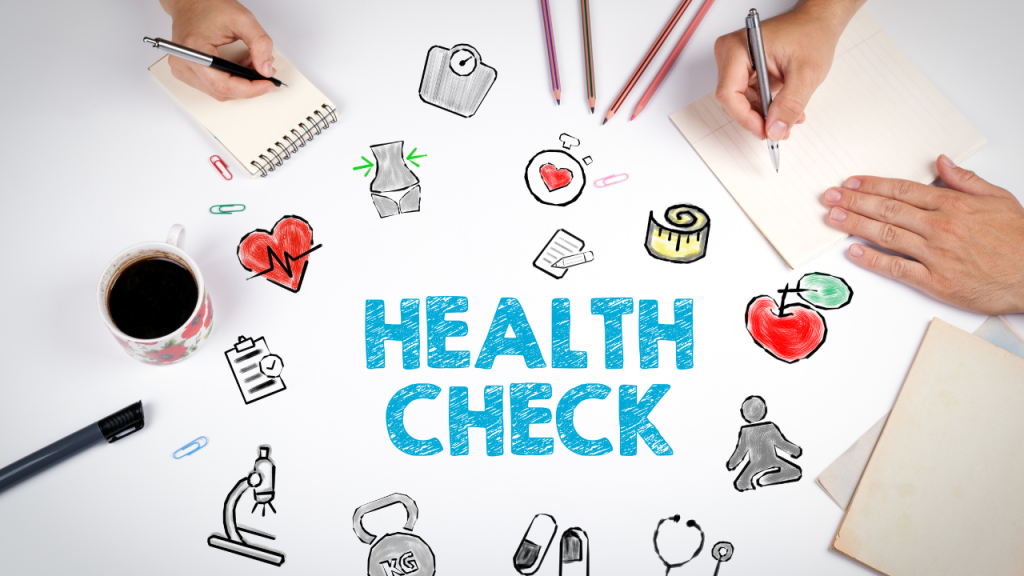
Future Prospects of Machine Intelligence
The future of machine intelligence holds immense possibilities. Continued advancements in machine learning, coupled with interdisciplinary research, will lead to breakthroughs in areas such as natural language understanding, robotics, and cognitive computing. To ensure the ethical and inclusive development of intelligent machines, it is crucial to address societal and ethical issues.
Conclusion
The question of whether we can make a machine intelligent is a complex one. While machines have made remarkable strides in simulating human-like intelligence, they are still far from replicating the full spectrum of human cognitive abilities. However, with ongoing research and advancements, the boundaries of machine intelligence continue to expand. It is crucial to navigate the challenges and ethical considerations associated with this technology to harness its potential for the benefit of humanity.