Exploring the History and Evolution of AI
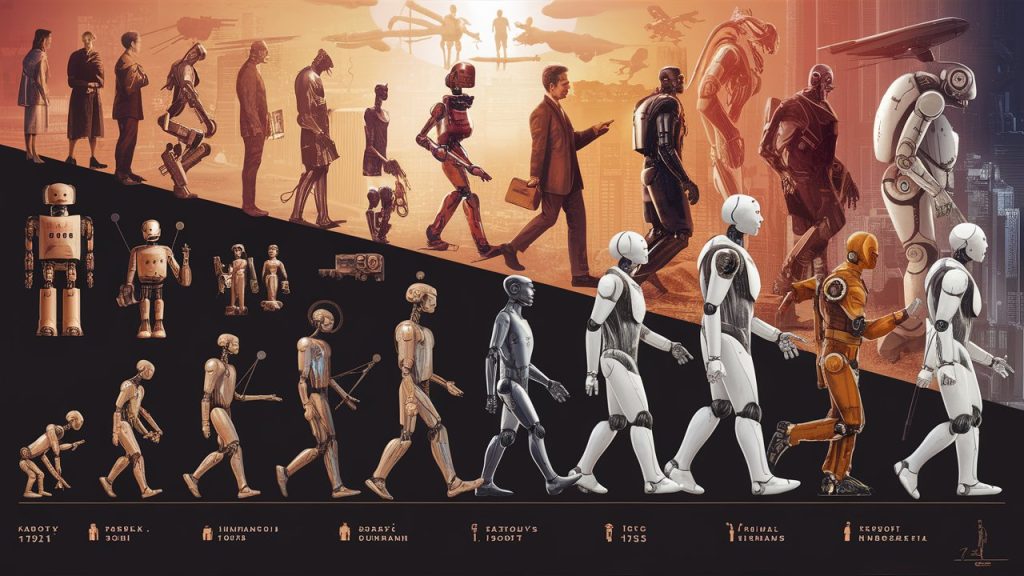
Artificial intelligence is a specialty within computer science that is concerned with creating systems that can replicate human intelligence and problem-solving abilities. They do this by taking in a myriad of data, processing it, and learning from their past in order to streamline and improve in the future. A normal computer program would need human interference in order to fix bugs and improve processes. The History of Artificial Intelligence: The idea of “artificial intelligence” goes back thousands of years, to ancient philosophers considering questions of life and death. In ancient times, inventors made things called “automatons” which were mechanical and moved independently of human intervention. The word “automaton” comes from ancient Greek, and means “acting of one’s own will.” One of the earliest records of an automaton comes from 400 BCE and refers to a mechanical pigeon created by a friend of the philosopher Plato. Many years later, one of the most famous automatons was created by Leonardo da Vinci around the year 1495. So while the idea of a machine being able to function on its own is ancient, for the purposes of this article, we’re going to focus on the 20th century, when engineers and scientists began to make strides toward our modern-day AI. Groundwork for AI 1900-1950: In the early 1900s, there was a lot of media created that centered around the idea of artificial humans. So much so that scientists of all sorts started asking the question: is it possible to create an artificial brain? Some creators even made some versions of what we now call “robots” (and the word was coined in a Czech play in 1921) though most of them were relatively simple. These were steam-powered for the most part, and some could make facial expressions and even walk. Notable Dates Birth of AI: 1950-1956 This range of time was when the interest in AI really came to a head. Alan Turing published his work “Computer Machinery and Intelligence” which eventually became The Turing Test, which experts used to measure computer intelligence. The term “artificial intelligence” was coined and came into popular use. Notable Dates AI Maturation: 1957-1979 The time between when the phrase “artificial intelligence” was created, and the 1980s was a period of both rapid growth and struggle for AI research. The late 1950s through the 1960s was a time of creation. From programming languages that are still in use to this day to books and films that explored the idea of robots, AI became a mainstream idea quickly. The 1970s showed similar improvements, such as the first anthropomorphic robot being built in Japan, to the first example of an autonomous vehicle being built by an engineering grad student. However, it was also a time of struggle for AI research, as the U.S. government showed little interest in continuing to fund AI research. AI Boom: 1980-1987 Most of the 1980s showed a period of rapid growth and interest in AI, now labeled as the “AI boom.” This came from both breakthroughs in research, and additional government funding to support the researchers. Deep Learning techniques and the use of Expert System became more popular, both of which allowed computers to learn from their mistakes and make independent decisions. Notable Dates AI Winter: 1987-1993 As the AAAI warned, an AI Winter came. The term describes a period of low consumer, public, and private interest in AI which leads to decreased research funding, which, in turn, leads to few breakthroughs. Both private investors and the government lost interest in AI and halted their funding due to high cost versus seemingly low return. This AI Winter came about because of some setbacks in the machine market and expert systems, including the end of the Fifth Generation project, cutbacks in strategic computing initiatives, and a slowdown in the deployment of expert systems. AI Agents: 1993-2011 Early 90s showed some impressive strides forward in AI research, including the introduction of the first AI system that could beat a reigning world champion chess player. This era also introduced AI into everyday life via innovations such as the first Roomba and the first commercially-available speech recognition software on Windows computers. The surge in interest was followed by a surge in funding for research, which allowed even more progress to be made. Notable Dates Artificial Intelligence is Everywhere: 2012 – Present That brings us to the most recent developments in AI, up to the present day. We’ve seen a surge in common-use AI tools, such as virtual assistants, search engines, etc. This time period also popularized Deep Learning and Big Data. Notable Dates Generative AI: Generative AI made significant strides in 2023, with the emergence of various models like Meta’s LLaMA 2, Google’s Bard chatbot, Baidu’s Ernie Bot, and OpenAI’s GPT-4. Despite initial hype, the year saw a focus on understanding the limitations and potential of generative AI, aiming to integrate it into practical applications for productivity enhancement. Augmented Reality (AR) and Quantum Computing: Alongside AI, other technologies like AR and quantum computing also saw significant advancements in 2023. AR technologies, such as Apple’s Vision Pro headset, and quantum computing developments like IBM’s System Two and Heron quantum chip, marked notable progress in their respective fields, hinting at a future where these technologies could play a more integrated role in everyday life Examples of AI Systems The global artificial intelligence market is over $136.6 billion, while the AI industry is expected to grow by 13x in the next seven years with a CAGR (compound annual growth rate) of 38.1%. Another report shows that 87% of global companies think AI gives them a competitive edge in the market. Be it in our personal or professional life, we cannot avoid using AI products in some form. AI has already made a positive impact across a broad range of industries. It can automate processes to free employees of unnecessary labor, provide personalized learning options for students, enable cybersecurity companies to deploy faster solutions and help fashion companies design better-fitting clothing for their customers.